High-dimensional data feature selection method based on graph neural network and spectral clustering
A feature selection method and neural network technology, applied in the field of high-dimensional data feature selection, can solve problems such as focusing on model results and ignoring interaction relationships
- Summary
- Abstract
- Description
- Claims
- Application Information
AI Technical Summary
Problems solved by technology
Method used
Image
Examples
Embodiment Construction
[0061] The invention will be further described below in conjunction with the accompanying drawings and specific implementation examples. In the present invention, each gene is used as a node to establish a gene relationship graph structure model, and the gene relationship data is added to the gene relationship graph as side information. It should be noted that there are many gene relationships, such as homologous expression Co-expression, Physical Interaction and Pathway, etc., taking Physical Interaction as an example of the edge relationship, the graph structure model constructed is an undirected graph. In the gene relationship graph, each node represents each gene and score, and each The edges represent the mutual relationship between every two genes, and the nodes are divided into two categories in this method: isolated nodes and normal nodes. Genes not involved in prior knowledge will form isolated nodes during the establishment of the graph structure, but isolated nodes ...
PUM
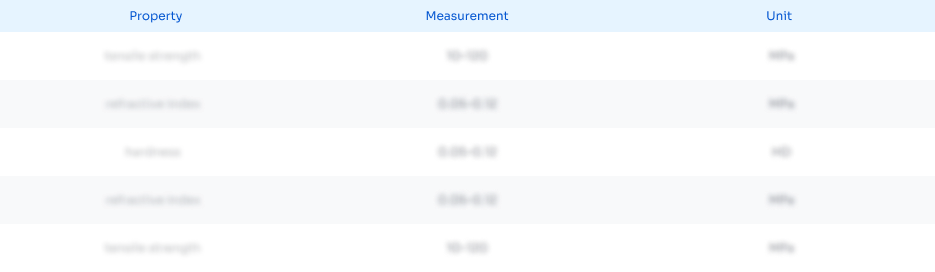
Abstract
Description
Claims
Application Information
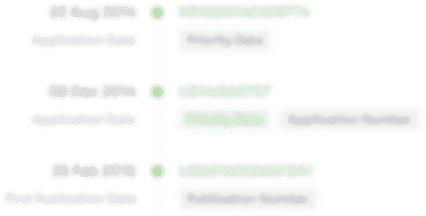
- R&D
- Intellectual Property
- Life Sciences
- Materials
- Tech Scout
- Unparalleled Data Quality
- Higher Quality Content
- 60% Fewer Hallucinations
Browse by: Latest US Patents, China's latest patents, Technical Efficacy Thesaurus, Application Domain, Technology Topic, Popular Technical Reports.
© 2025 PatSnap. All rights reserved.Legal|Privacy policy|Modern Slavery Act Transparency Statement|Sitemap|About US| Contact US: help@patsnap.com