Fault diagnosis methodbased on wavelet convolutional neural network
A convolutional neural network and fault diagnosis technology, which is applied to biological neural network models, neural architectures, instruments, etc., can solve problems such as long time consumption, decreased diagnosis rate, and insufficient integration, so as to improve feature extraction capabilities and reduce calculations. , Improving the performance of fault detection and diagnosis
- Summary
- Abstract
- Description
- Claims
- Application Information
AI Technical Summary
Problems solved by technology
Method used
Image
Examples
Embodiment Construction
[0039] The technical solutions of the present invention will be described in further detail below in conjunction with the accompanying drawings and specific embodiments.
[0040] A kind of fault diagnosis method based on wavelet convolutional neural network of the present invention has realized the multi-model dynamic monitoring of convolutional neural network (CNN) based on wavelet transform, and concrete process comprises the following steps:
[0041] Step 1: Filter the collected chemical data according to the equipment variables, divide them according to the equipment the variables belong to and the equipment the variables act on, and select the variables that are more independent and have a greater impact on the operation of the equipment, for example, the R301 reactor equipment The relevant variables are extracted, standardized and matrixed, and the variables of each period form a data matrix, which is used as the input of the wavelet transform algorithm and the convolutio...
PUM
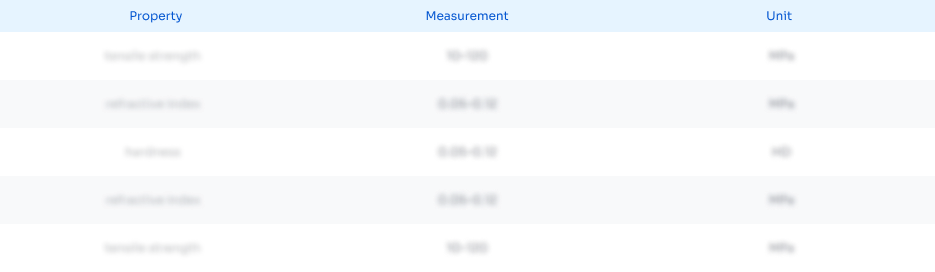
Abstract
Description
Claims
Application Information
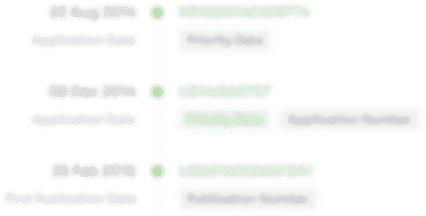
- R&D
- Intellectual Property
- Life Sciences
- Materials
- Tech Scout
- Unparalleled Data Quality
- Higher Quality Content
- 60% Fewer Hallucinations
Browse by: Latest US Patents, China's latest patents, Technical Efficacy Thesaurus, Application Domain, Technology Topic, Popular Technical Reports.
© 2025 PatSnap. All rights reserved.Legal|Privacy policy|Modern Slavery Act Transparency Statement|Sitemap|About US| Contact US: help@patsnap.com