Aero-engine remaining service life estimation method based on deep reinforcement learning
An aero-engine and reinforcement learning technology, which is applied in neural learning methods, machine learning, computer-aided design, etc., can solve problems such as overfitting and performance defects, and achieve strong generalization and accurate estimation of remaining service life.
- Summary
- Abstract
- Description
- Claims
- Application Information
AI Technical Summary
Problems solved by technology
Method used
Image
Examples
Embodiment Construction
[0045] The present embodiment adopts the status monitoring data of aero-engine sensors, which are collected by 21 sensors and mainly include: total temperature at the fan inlet, total temperature at the outlet of the low-pressure compressor, total temperature at the outlet of the high-pressure compressor, total temperature at the outlet of the low-pressure turbine, fan Inlet pressure, total pressure of external duct, total pressure of high pressure compressor outlet, fan physical speed, core machine physical speed, engine pressure ratio, high pressure compressor outlet static pressure, ratio of fuel consumption rate to high pressure compressor outlet static pressure, correction Fan speed, corrected core machine speed, outside culvert ratio, combustion chamber oil-air ratio, extraction enthalpy, rated fan speed, rated corrected fan speed, high-pressure turbine cooling air extraction volume, low-pressure turbine cooling air extraction volume.
[0046] The original sensor data set...
PUM
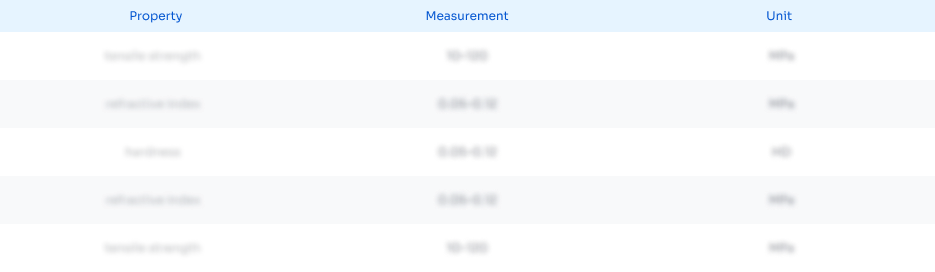
Abstract
Description
Claims
Application Information
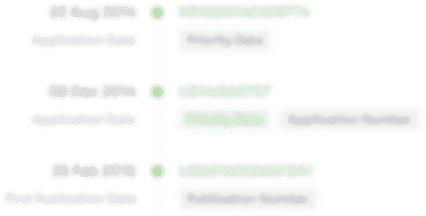
- R&D Engineer
- R&D Manager
- IP Professional
- Industry Leading Data Capabilities
- Powerful AI technology
- Patent DNA Extraction
Browse by: Latest US Patents, China's latest patents, Technical Efficacy Thesaurus, Application Domain, Technology Topic.
© 2024 PatSnap. All rights reserved.Legal|Privacy policy|Modern Slavery Act Transparency Statement|Sitemap