Data flow concept drift detection method based on historical model diversity
A concept drift and historical model technology, applied in the field of big data and machine learning, can solve the problems of unstable underlying distribution, and achieve the effect of real-time monitoring and response
- Summary
- Abstract
- Description
- Claims
- Application Information
AI Technical Summary
Problems solved by technology
Method used
Image
Examples
Embodiment 1
[0037] The online learning model based on the data stream extraction of intelligent production line based on industrial big data realizes the dynamic response mechanism of fault diagnosis and real-time monitoring and detection of gradual faults and sudden faults.
[0038] In this embodiment, the data flow concept drift detection method based on the diversity of historical models, the flow chart is as follows figure 1 As shown, the method includes the following steps:
[0039] S1 uses the online bagging method to process the data stream online;
[0040] S2 builds a base tree that retains historical diversity for building random forests;
[0041] S3 identifies concept drift areas and performs noise reduction processing;
[0042] S4 uses ensemble methods for concept drift detection;
[0043] S5 removes the maximum difference model and maintains the detection system update.
[0044] In step S1, the online bagging algorithm adopted is as follows. Given a training data set d=(x,...
PUM
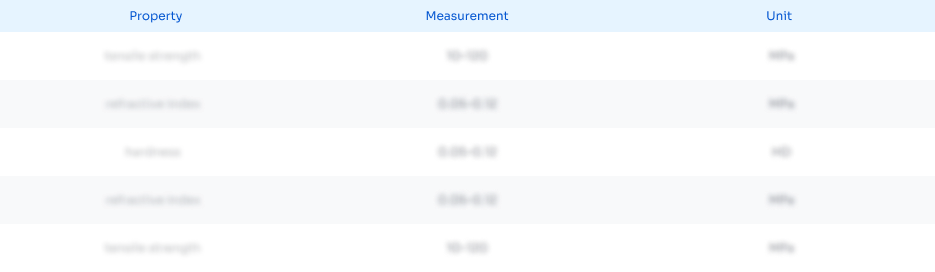
Abstract
Description
Claims
Application Information
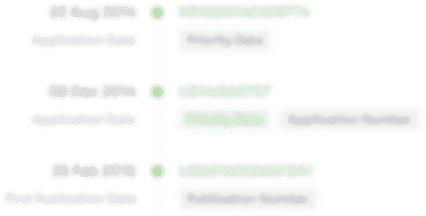
- R&D Engineer
- R&D Manager
- IP Professional
- Industry Leading Data Capabilities
- Powerful AI technology
- Patent DNA Extraction
Browse by: Latest US Patents, China's latest patents, Technical Efficacy Thesaurus, Application Domain, Technology Topic, Popular Technical Reports.
© 2024 PatSnap. All rights reserved.Legal|Privacy policy|Modern Slavery Act Transparency Statement|Sitemap|About US| Contact US: help@patsnap.com