New-drilled well workload prediction method based on ensemble learning
A workload and drilling technology, which is applied in the fields of geophysical exploration and artificial intelligence, can solve problems such as one-sided mapping of new drilling workload, fixed parameters, and lack of universality, so as to improve stability and operating speed, improve accuracy, The effect of reducing negative effects
- Summary
- Abstract
- Description
- Claims
- Application Information
AI Technical Summary
Problems solved by technology
Method used
Image
Examples
Embodiment Construction
[0022]Combine belowfigure 1 To further describe the present invention in detail:
[0023]A. Construct historical data set of reservoir development:
[0024]Extract oil field geological data and real-time oil well reserve data from seismic database and oil reservoir history database, map the oil field geological data and real-time oil well reserve data to the same data source, and use the five-year number of new wells adjusted by the history of the oil field block as the new drilling Workload prediction standard, after preprocessing, obtain the historical data set of reservoir development.
[0025]B. Using particle swarm optimization method to optimize the key hyperparameter combination:
[0026](1) Initial model construction
[0027]Build a new drilling workload prediction initial model based on random forest, select the maximum feature number of a single decision tree, the number of subtrees and the minimum sample leaf size as key hyperparameter combinations, and randomly select appropriate data ...
PUM
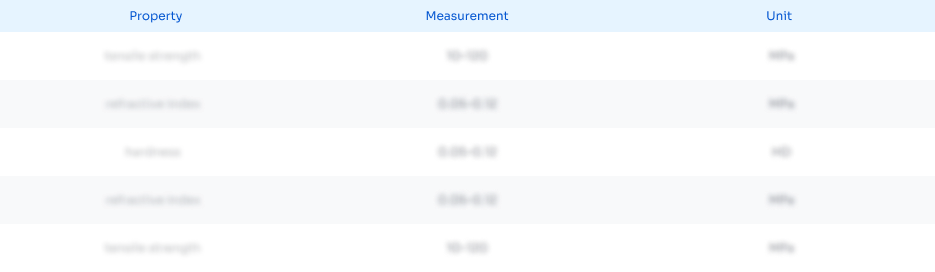
Abstract
Description
Claims
Application Information
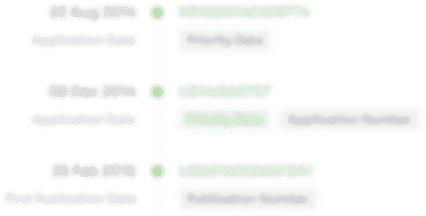
- R&D
- Intellectual Property
- Life Sciences
- Materials
- Tech Scout
- Unparalleled Data Quality
- Higher Quality Content
- 60% Fewer Hallucinations
Browse by: Latest US Patents, China's latest patents, Technical Efficacy Thesaurus, Application Domain, Technology Topic, Popular Technical Reports.
© 2025 PatSnap. All rights reserved.Legal|Privacy policy|Modern Slavery Act Transparency Statement|Sitemap|About US| Contact US: help@patsnap.com