Convolutional long short-term memory network space-time sequence prediction method improved by utilizing attention mechanism
A long-short-term memory and sequence prediction technology, applied in neural learning methods, biological neural network models, neural architectures, etc., can solve high-dimensional non-computable problems, achieve the effect of reducing parameters, high accuracy, and improving efficiency
- Summary
- Abstract
- Description
- Claims
- Application Information
AI Technical Summary
Problems solved by technology
Method used
Image
Examples
Embodiment Construction
[0055] In order to illustrate the technical solution of the present invention more clearly, the technical solution of the present invention is described in further detail below in conjunction with the accompanying drawings:
[0056] Such as figure 1 Described; A method for predicting spatio-temporal sequences using an improved convolutional long-term short-term memory network (Convolutional LSTM) using the attention (Attention) mechanism,
[0057] Among them, the attention (Attention) mechanism refers to: the Attention mechanism imitates the characteristics of human beings when observing things, focuses attention on key target information, and assigns different weights to different information through a special alignment model, so as to achieve emphasis. important information and suppress unimportant information.
[0058] The Convolutional LSTM time-space sequence: the Convolutional LSTM here refers to the structure of a long-term short-term memory network (LSTM) that adopts ...
PUM
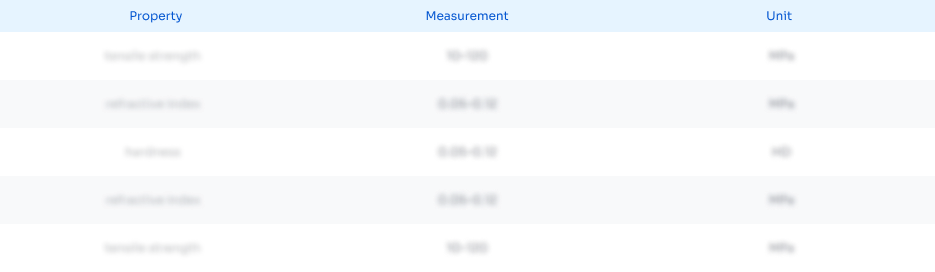
Abstract
Description
Claims
Application Information
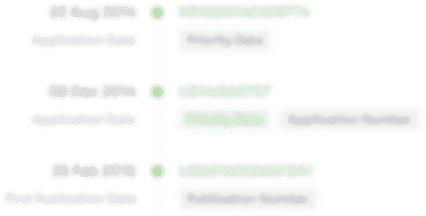
- R&D Engineer
- R&D Manager
- IP Professional
- Industry Leading Data Capabilities
- Powerful AI technology
- Patent DNA Extraction
Browse by: Latest US Patents, China's latest patents, Technical Efficacy Thesaurus, Application Domain, Technology Topic, Popular Technical Reports.
© 2024 PatSnap. All rights reserved.Legal|Privacy policy|Modern Slavery Act Transparency Statement|Sitemap|About US| Contact US: help@patsnap.com