Upper pull rod fault detection method based on deep learning
A technology of fault detection and deep learning, applied in the direction of railway vehicle shape measuring devices, biological neural network models, instruments, etc., can solve the problems of missed detection detection efficiency, error-prone upper pull rods, etc., to improve detection accuracy, improve quality and The effect of detection efficiency and labor cost saving
- Summary
- Abstract
- Description
- Claims
- Application Information
AI Technical Summary
Problems solved by technology
Method used
Image
Examples
specific Embodiment approach 1
[0028] DETAILED DESCRIPTION OF THE PREFERRED EMBODIMENTS 1. This implementation will be described with reference to FIG. 1 . A method for detecting a pull-up rod fault based on deep learning in this embodiment, the method is specifically implemented through the following steps:
[0029] Step 1, collect the train image to be detected, and obtain the image of the region of interest from the collected train image;
[0030] Set up high-definition equipment at the bottom of the railway freight car tracks to take pictures of trains passing at high speed and obtain high-definition images of the bottom of the car body. Using line scanning, seamless stitching of images can be realized, and a two-dimensional image with a large field of view and high precision can be generated. According to the wheelbase information, bogie type and vehicle type of the EMU, the upper tie rod is roughly positioned, and the local area image containing the upper tie rod parts is cut out from the captured pi...
specific Embodiment approach 2
[0037] Specific embodiment two: the difference between this embodiment and specific embodiment one is: in step six, the FasterRCNN model that contains the image to be recognized that is extracted in step five and includes the upper tie rod is input into the trained FasterRCNN model, if the FasterRCNN model detects the upper tie rod joint and For the position of the flat iron, determine whether the upper tie rod has fallen off according to the connection status of the upper tie rod joint and the flat iron;
[0038] If there is a failure of the upper tie rod falling off, a message will be generated and uploaded to the alarm platform; if there is no failure of the upper tie rod falling off, the image to be recognized (that is, the image after enhanced processing) will be input into the trained Unet semantic segmentation model, and the trained Unet semantic segmentation model will be used. The Unet semantic segmentation model segmented the upper tie rod joint and the upper tie rod ...
specific Embodiment approach 3
[0039] Embodiment 3: The difference between this embodiment and Embodiment 1 is that in step 2, the image of the region of interest is enhanced, and the image of the region of interest after the enhancement is obtained. The specific process is as follows:
[0040]
[0041]
[0042]
[0043] Among them, v(x, y) represents the gray value of the pixel point (x, y) in the image of the region of interest, and I 2 (x, y) represents the gray value of the pixel point (x, y) of the image of the region of interest after nonlinear transformation, Represents the average gray value of all pixels in the image of the region of interest, m(x, y) and kv(x, y)) are intermediate variables, and a is the adjustment coefficient;
[0044] The smaller a, the larger the gray value of pixels with small gray values after nonlinear transformation, but at the same time it is easy to lose texture detail information; the larger a is, although the texture information is retained, but the pixels w...
PUM
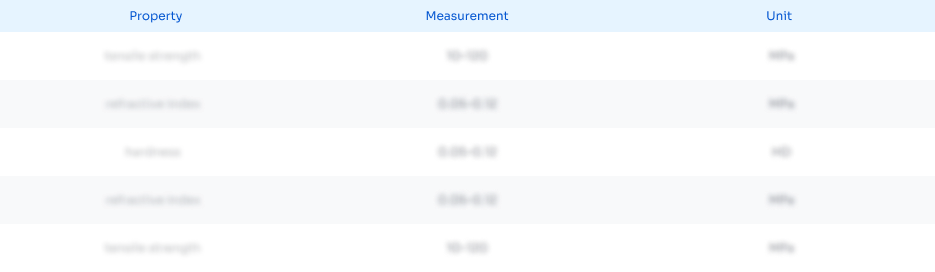
Abstract
Description
Claims
Application Information
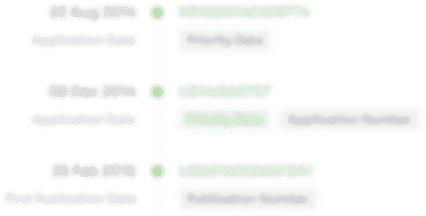
- R&D Engineer
- R&D Manager
- IP Professional
- Industry Leading Data Capabilities
- Powerful AI technology
- Patent DNA Extraction
Browse by: Latest US Patents, China's latest patents, Technical Efficacy Thesaurus, Application Domain, Technology Topic, Popular Technical Reports.
© 2024 PatSnap. All rights reserved.Legal|Privacy policy|Modern Slavery Act Transparency Statement|Sitemap|About US| Contact US: help@patsnap.com