Handwritten picture classification method based on quantum self-learning and self-training network
A picture classification and self-training technology, applied in neural learning methods, biological neural network models, instruments, etc., can solve the problems of cumbersome training, small search range, and inability to guarantee the reliability and stability of classification results, so as to improve accuracy, The effect of a wide search space
- Summary
- Abstract
- Description
- Claims
- Application Information
AI Technical Summary
Problems solved by technology
Method used
Image
Examples
Embodiment Construction
[0036] The present invention will be further described below in conjunction with the accompanying drawings.
[0037] Refer to attached figure 1 , to further describe in detail the specific steps of the present invention.
[0038] Step 1, construct the neural network.
[0039] Build a neural network with a structure of an input layer, an output layer, and at least four hidden layers connected sequentially between the input layer and the output layer. The input layer contains 784 neuron nodes, and the output layer contains There are 10 neuron nodes, and each hidden layer contains 5 neuron nodes.
[0040] Step 2, generate a training set.
[0041] Select at least 56,000 handwritten pictures containing 10 categories, scale each picture to 28×28 pixels to form a one-dimensional vector, and form all one-dimensional vectors into a training set.
[0042] Step 3, according to the following formula, calculate the length of the quantum chromosome individual:
[0043]
[0044]Among...
PUM
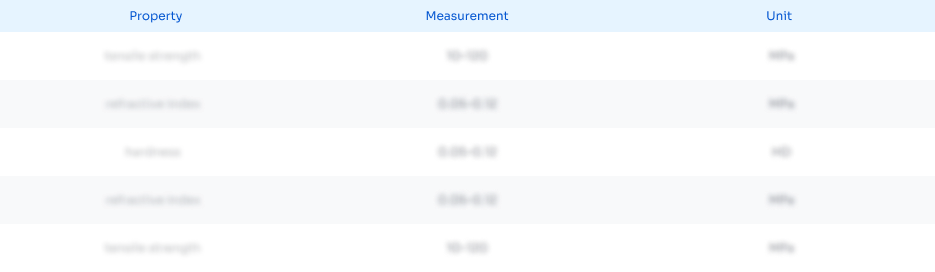
Abstract
Description
Claims
Application Information
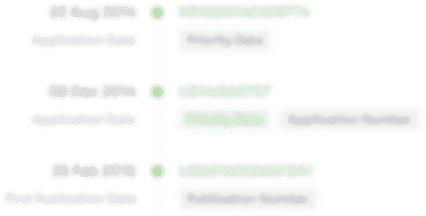
- R&D Engineer
- R&D Manager
- IP Professional
- Industry Leading Data Capabilities
- Powerful AI technology
- Patent DNA Extraction
Browse by: Latest US Patents, China's latest patents, Technical Efficacy Thesaurus, Application Domain, Technology Topic, Popular Technical Reports.
© 2024 PatSnap. All rights reserved.Legal|Privacy policy|Modern Slavery Act Transparency Statement|Sitemap|About US| Contact US: help@patsnap.com