Multi-feature fusion image classification method based on deep learning
A multi-feature fusion and deep learning technology, applied in neural learning methods, image analysis, image enhancement, etc., can solve the problems of inaccurate feature extraction and classification accuracy, and achieve the effect of improving classification accuracy and feature accuracy
- Summary
- Abstract
- Description
- Claims
- Application Information
AI Technical Summary
Problems solved by technology
Method used
Image
Examples
Embodiment Construction
[0026] Below in conjunction with accompanying drawing, the present invention will be further explained;
[0027] The hardware environment of this embodiment is 8vCPU / 64G memory, the GPU is V100, and the software operating environment is CUDA:9.2.148, python3.7, pytorch 1.0.1.post2.
[0028] Such as figure 1 As shown, the classification steps of a multi-feature fusion image classification method based on deep learning are as follows:
[0029] Step 1. Divide the collected digital pathological images of eye tumors into a training set, a verification set and a test set, and each set contains three types of samples: early stage, middle stage, and late stage.
[0030] Step 2. Normalize the pictures in the training set, verification set and test set and then cut them to 224*224, and randomly flip the pictures in the training set horizontally, vertically, and modify the brightness according to the probability P1=0.5 Flips the image horizontally.
[0031] Step 3, establish as figu...
PUM
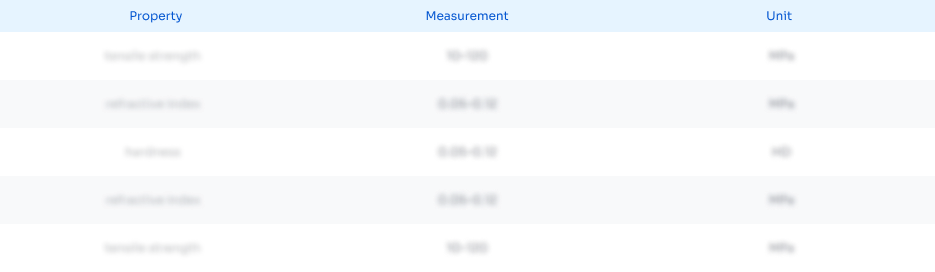
Abstract
Description
Claims
Application Information
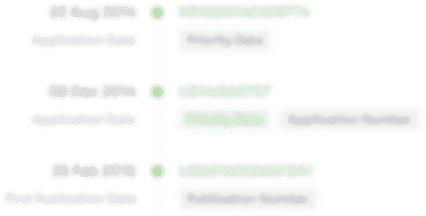
- R&D Engineer
- R&D Manager
- IP Professional
- Industry Leading Data Capabilities
- Powerful AI technology
- Patent DNA Extraction
Browse by: Latest US Patents, China's latest patents, Technical Efficacy Thesaurus, Application Domain, Technology Topic, Popular Technical Reports.
© 2024 PatSnap. All rights reserved.Legal|Privacy policy|Modern Slavery Act Transparency Statement|Sitemap|About US| Contact US: help@patsnap.com