Compressed multi-scale feature fusion network-based image super-resolution reconstruction method
A multi-scale feature fusion network technology, applied in image super-resolution reconstruction, image super-resolution reconstruction based on compressed multi-scale feature fusion network, can solve high-resolution image peak signal-to-noise ratio and low structural similarity problem, to achieve the effect of improving the peak signal-to-noise ratio and structural similarity, increasing the number, and improving the fitting ability
- Summary
- Abstract
- Description
- Claims
- Application Information
AI Technical Summary
Problems solved by technology
Method used
Image
Examples
Embodiment Construction
[0034] specific implementation plan
[0035] Below in conjunction with accompanying drawing and specific embodiment, the present invention is described in further detail:
[0036] refer to figure 1 , an image super-resolution reconstruction method based on compressed multi-scale feature fusion network, including the following steps:
[0037] Step 1) Get the training sample set:
[0038]Step 1a) Extract 291 RGB images from the Berkeley segmentation database, and rotate each image, the degrees of rotation are 0°, 90°, 180°, and 270°, and then perform scale transformation on each image, and the transformation scales are respectively 1 times, 6 times, 7 times, 8 times, 9 times, get 5820 RGB images;
[0039] Step 1b) Perform format conversion on 5820 RGB images, and extract a Y-channel image from each of the obtained 5820 YCbCr images to obtain 5820 Y-channel images, and number the 5820 Y-channel images to obtain 5820 images with Numbered Y-channel images;
[0040] Step 1c) Pe...
PUM
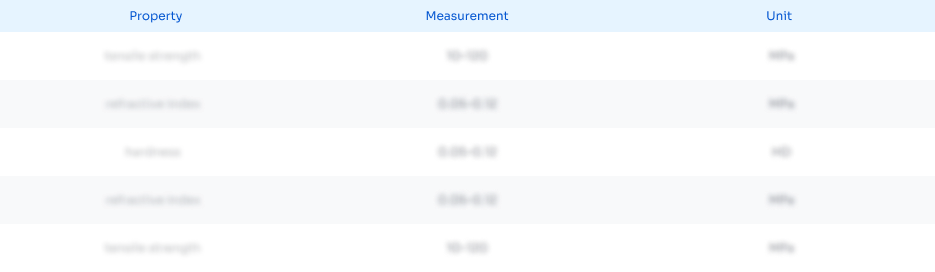
Abstract
Description
Claims
Application Information
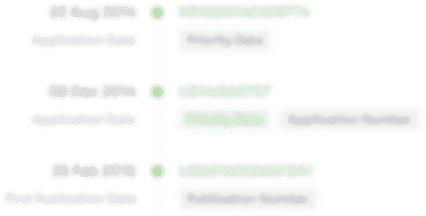
- R&D Engineer
- R&D Manager
- IP Professional
- Industry Leading Data Capabilities
- Powerful AI technology
- Patent DNA Extraction
Browse by: Latest US Patents, China's latest patents, Technical Efficacy Thesaurus, Application Domain, Technology Topic, Popular Technical Reports.
© 2024 PatSnap. All rights reserved.Legal|Privacy policy|Modern Slavery Act Transparency Statement|Sitemap|About US| Contact US: help@patsnap.com