Image coloring method based on improved deep separable convolutional neural network
A convolutional neural network and image coloring technology, applied in the field of image processing technology and deep learning, can solve the problems of memory consumption and memory cost increase, restricting industrial applications, and low network computing efficiency, so as to reduce the amount of parameters and improve coloring Accuracy, the effect of reducing the number of parameter calculations
- Summary
- Abstract
- Description
- Claims
- Application Information
AI Technical Summary
Problems solved by technology
Method used
Image
Examples
Embodiment Construction
[0024] Image coloring methods based on deep learning have achieved certain results in recent years, but most of them require a lot of computing resources and computing time, making it difficult to deploy on mobile or embedded devices. The present invention proposes an efficient method using a small number of parameters while substantially not affecting the coloring effect. The network structure of the present invention comprehensively considers global semantic features and local pixel features, and uses methods such as residual, depth-separable convolution, and channel weighting to reduce parameters and improve performance.
[0025] combine figure 1 , an image colorization method based on an improved depthwise separable convolutional neural network, including the following steps:
[0026] Step 1, construct an image dataset;
[0027] Step 2, constructing an improved depthwise separable convolutional coloring neural network; combined with figure 2 , the construction process ...
PUM
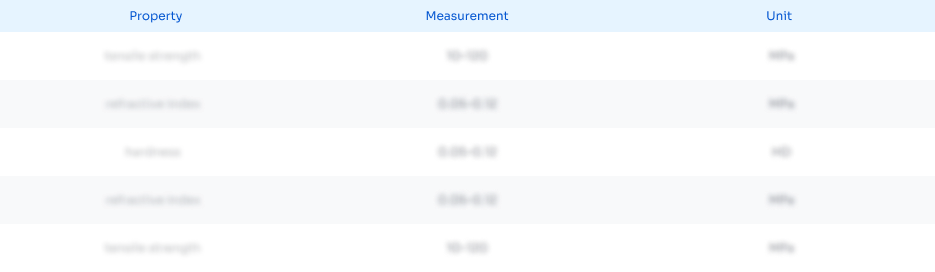
Abstract
Description
Claims
Application Information
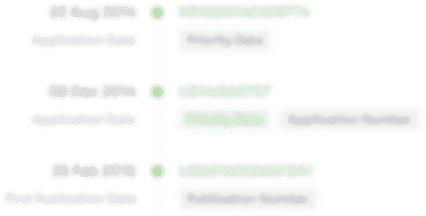
- R&D Engineer
- R&D Manager
- IP Professional
- Industry Leading Data Capabilities
- Powerful AI technology
- Patent DNA Extraction
Browse by: Latest US Patents, China's latest patents, Technical Efficacy Thesaurus, Application Domain, Technology Topic, Popular Technical Reports.
© 2024 PatSnap. All rights reserved.Legal|Privacy policy|Modern Slavery Act Transparency Statement|Sitemap|About US| Contact US: help@patsnap.com