3D human motion prediction method based on depth state space model
A technology of state space model and human motion, applied in biological neural network model, character and pattern recognition, image data processing, etc., can solve the problems that affect the later time prediction and cannot realize the prediction
- Summary
- Abstract
- Description
- Claims
- Application Information
AI Technical Summary
Problems solved by technology
Method used
Image
Examples
experiment example
[0081]1.1 Data sets and experiment details
[0082](1) Data set
[0083]Human3.6m (H3.6M): H3.6M is the most commonly used data set in human motion prediction. The data set consists of 15 actions implemented by seven professional actors, such as walk, eat, smoking, and discussions.
[0084]3D POSE IN The Wild DataSet (3DPW): 3DPW is an field dataset with exact 3D posture, including a variety of human behavior, such as shopping, sports, etc. The data set includes 60 posture sequences, more than 51 kV.
[0085](2) Experimental details
[0086]In the experiment, all experimental settings and data processing consistent with the baseline. The model of the present invention is implemented based on Tensorflow. The mean per joint positionerror, MPJPE) of the average unit of millimeters is the metric index of the present invention. All models are trained in the ADAM optimizer, and the learning rate is initialized to 0.0001. Super parameter λ1: λ2Set to 3: 1.
[0087]1.2 and advanced methods
[0088]Baseline: (1)...
PUM
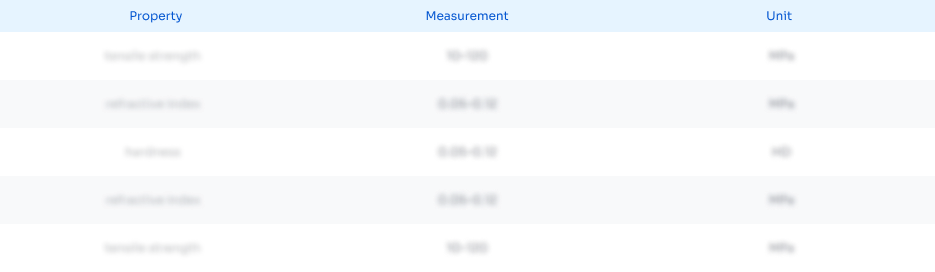
Abstract
Description
Claims
Application Information
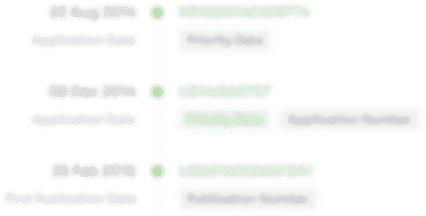
- R&D
- Intellectual Property
- Life Sciences
- Materials
- Tech Scout
- Unparalleled Data Quality
- Higher Quality Content
- 60% Fewer Hallucinations
Browse by: Latest US Patents, China's latest patents, Technical Efficacy Thesaurus, Application Domain, Technology Topic, Popular Technical Reports.
© 2025 PatSnap. All rights reserved.Legal|Privacy policy|Modern Slavery Act Transparency Statement|Sitemap|About US| Contact US: help@patsnap.com