Brain network classification method combining node attributes and multi-level topology
A classification method and brain network technology, applied in the field of brain network classification combining node attributes and multi-level topology, can solve the problems of improving, limiting classification performance, failing to fully consider the multi-level topology characteristics of nodes in brain networks, etc. The effect of classification performance
- Summary
- Abstract
- Description
- Claims
- Application Information
AI Technical Summary
Problems solved by technology
Method used
Image
Examples
Embodiment
[0045] see figure 1, the present invention provides a technical solution: a brain network classification method combining node attributes and multi-level topology, comprising: ① first preprocessing functional Magnetic Resonance Imaging (fMRI) data, using automated anatomy The Automated Anatomical Labeling (AAL) template was used to generate the functional connectivity matrix of the whole brain network, and the DMN was used as the region of interest to construct an unbiased brain network using the Kruskal algorithm. ② Extract the betweenness of brain region nodes on the unbiased brain network as a local attribute, and use the two-sample t-test method to extract the characteristics of brain regions with significant differences between groups. ③Use the sub-network kernel to extract multi-level topological features on the unbiased brain network, generate the sub-network kernel matrix, use Kernel Principal Component Analysis (KPCA) to extract the optimal topological features, and f...
PUM
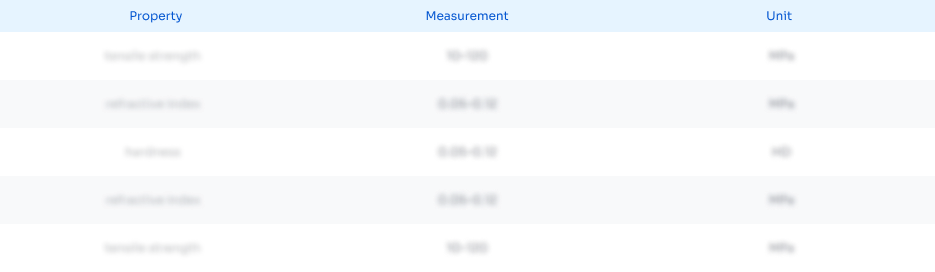
Abstract
Description
Claims
Application Information
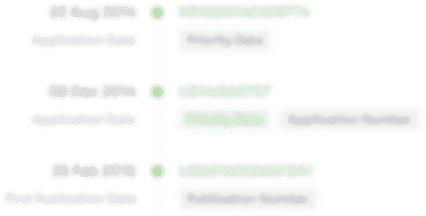
- R&D
- Intellectual Property
- Life Sciences
- Materials
- Tech Scout
- Unparalleled Data Quality
- Higher Quality Content
- 60% Fewer Hallucinations
Browse by: Latest US Patents, China's latest patents, Technical Efficacy Thesaurus, Application Domain, Technology Topic, Popular Technical Reports.
© 2025 PatSnap. All rights reserved.Legal|Privacy policy|Modern Slavery Act Transparency Statement|Sitemap|About US| Contact US: help@patsnap.com