Spatial non-cooperative target pose estimation method based on deep learning
A non-cooperative target and pose estimation technology, applied in neural learning methods, calculations, computer components, etc., can solve problems such as large attitude errors and few data sets, and achieve the effect of meeting real-time requirements
- Summary
- Abstract
- Description
- Claims
- Application Information
AI Technical Summary
Problems solved by technology
Method used
Image
Examples
Embodiment 1
[0087] In this embodiment, the space non-cooperative target is selected as a spacecraft with a known three-dimensional model, and the pose estimation of the target spacecraft is performed. The implementation steps are as follows:
[0088] (1) Divide the pose category intervals, and make training sets, verification sets, and test sets.
[0089] (1-1) Divide the attitude category interval: set the angle classification interval threshold δ=6°, and divide the attitude category at an interval of 6°. Estimated angle minimum θ min =-30°, estimated maximum angle θ max = 30°. Angle interval [-33°, -27°) is attitude category 0, angle interval [-27°, -21°) is attitude category 1, angle interval [-21°, -15°) is attitude category 2, angle interval [-15°, -9°) is attitude category 3, the angle interval [-9°, -3°) is attitude category 4, the angle interval [-3°, 3°) is attitude category 5, and the angle interval [3° ,9°) is attitude category 6, the angle interval [9°,15°) is attitude ca...
PUM
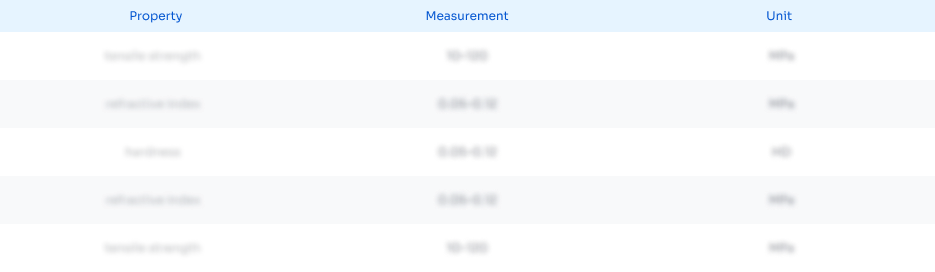
Abstract
Description
Claims
Application Information
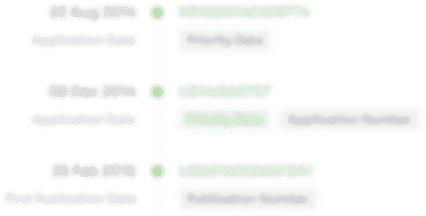
- R&D Engineer
- R&D Manager
- IP Professional
- Industry Leading Data Capabilities
- Powerful AI technology
- Patent DNA Extraction
Browse by: Latest US Patents, China's latest patents, Technical Efficacy Thesaurus, Application Domain, Technology Topic, Popular Technical Reports.
© 2024 PatSnap. All rights reserved.Legal|Privacy policy|Modern Slavery Act Transparency Statement|Sitemap|About US| Contact US: help@patsnap.com