Combinable weak authenticator-based named entity identification algorithm architecture
A technology of named entity recognition and entity recognition, applied in neural learning methods, instruments, biological neural network models, etc., can solve problems such as large differences in entity boundaries, errors and failures in named entity recognition, and achieve increased robustness and enriched training samples Effect
- Summary
- Abstract
- Description
- Claims
- Application Information
AI Technical Summary
Problems solved by technology
Method used
Image
Examples
Example Embodiment
[0127]Example 1
[0128]A algorithm architecture based on named entity identification based on a combined weak authenticator, including: entity identification part and result authentication section;
[0129]The entity identification portion is used to complete the identification task to obtain an identification result;
[0130]The result authentication portion includes two and more weak certificates, respectively, for verification of the identification result in each weak authenticator corresponding to the segmentation target.
[0131]The algorithm architecture also includes an information input layer, an entity identification layer, a data conversion layer, and a weak authenticator output layer;
[0132]The token information input layer is characterized by feature extraction: The layer includes a feature extraction module; after processing the text input and entity description input through the feature extraction module, as the first input information of the entity identification layer;
[0133]The ...
Example Embodiment
[0144]Example 2,
[0145]A algorithm architecture based on a nomenched entity identified as described in Example 1, the architecture further includes a training information flow, the training information flow specifically comprising: pre-training information flow and joint training information flow;
[0146]Among them, the pre-training information stream includes, entity identification module pre-training information flow, boundary weak certifier pre-training information flow and type weak certifier pre-training information;
[0147]Entity identification module pre-training information flow: The feature extraction module in the information input layer and the entity identification module in the entity identification layer participate in the training, the weak certification layer closes the input and output interface; the training data is the original training mark.
[0148]Boundary Weak Cautioner Module Predictive Information Flow: The Feature Extract Module in the Information Input layer and t...
Example Embodiment
[0151]Example 3,
[0152]As described in Example 1, an algorithm architecture based on a nomenched entity identified, the entity identification module includes a plurality of groups of neural networks, and the activation function of the neural network, preferably, each group of neural networks can be extracted sequences. The network structure of the feature, such as the Bi-LSTM neural network, Bi-GRU neural network, Bi-GRU neural network, or deep convolutional neural network.
[0153]Preferably, the feature extraction module is loaded with a pre-training language model based on self-focus mechanism; preferably, load the Bert algorithm.
[0154]The boundary weak authenticator module is loaded with neural networks (such as Bi-LSTM neural network, Bi-GRU neural network or deep convolutional neural network, etc.) and neural networks (such as SIGMOID or Softmax). ), Used to perform border legitimacy determination;
[0155]The type weak authenticator module is loaded with neural networks with extract...
PUM
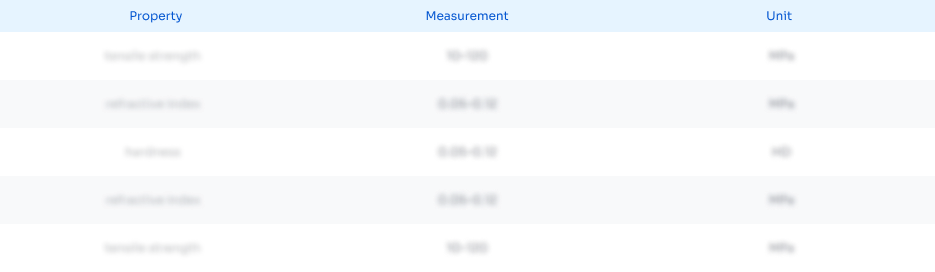
Abstract
Description
Claims
Application Information
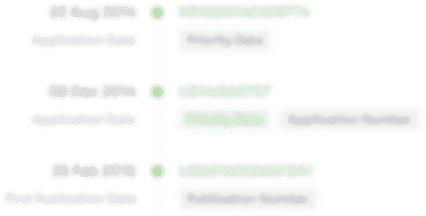
- R&D Engineer
- R&D Manager
- IP Professional
- Industry Leading Data Capabilities
- Powerful AI technology
- Patent DNA Extraction
Browse by: Latest US Patents, China's latest patents, Technical Efficacy Thesaurus, Application Domain, Technology Topic.
© 2024 PatSnap. All rights reserved.Legal|Privacy policy|Modern Slavery Act Transparency Statement|Sitemap