Power optimization method for autonomous navigation UAV based on deep reinforcement learning
A technology of reinforcement learning and autonomous navigation, applied to mechanical equipment, combustion engines, internal combustion piston engines, etc. Consumption performance and other issues, to achieve the effect of improving computing power utilization efficiency, increasing battery life, and improving power consumption utilization
- Summary
- Abstract
- Description
- Claims
- Application Information
AI Technical Summary
Problems solved by technology
Method used
Image
Examples
Embodiment Construction
[0040] The accompanying drawings are for illustrative purposes only, and should not be construed as limiting the present invention; in order to better illustrate this embodiment, certain components in the accompanying drawings will be omitted, enlarged or reduced, and do not represent the size of the actual product; for those skilled in the art It is understandable that some well-known structures and descriptions thereof may be omitted in the drawings. The positional relationship described in the drawings is for illustrative purposes only, and should not be construed as limiting the present invention.
[0041] This embodiment discloses a power optimization method for self-driving drones based on deep reinforcement learning. The method realizes autonomous navigation through a deep neural network, combines reinforcement learning, and infers power from the environment state of the drone. The optimal configuration improves the endurance of the drone. Specifically include the foll...
PUM
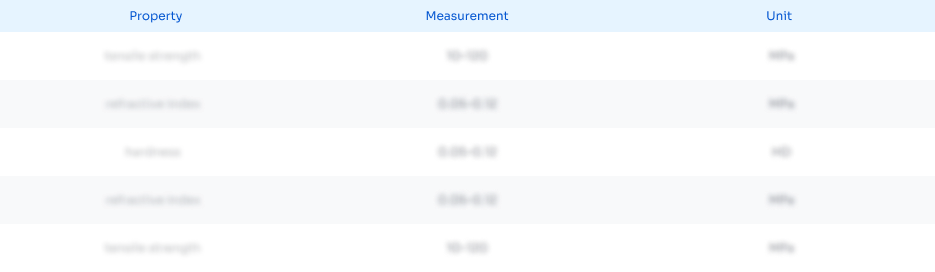
Abstract
Description
Claims
Application Information
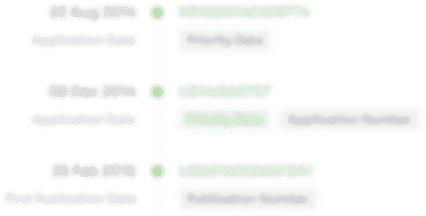
- R&D
- Intellectual Property
- Life Sciences
- Materials
- Tech Scout
- Unparalleled Data Quality
- Higher Quality Content
- 60% Fewer Hallucinations
Browse by: Latest US Patents, China's latest patents, Technical Efficacy Thesaurus, Application Domain, Technology Topic, Popular Technical Reports.
© 2025 PatSnap. All rights reserved.Legal|Privacy policy|Modern Slavery Act Transparency Statement|Sitemap|About US| Contact US: help@patsnap.com