Automatic driving safety scene meta-modeling method driven by spatio-temporal trajectory data
A spatio-temporal trajectory, autonomous driving technology, applied in structured data retrieval, electronic digital data processing, digital data information retrieval, etc., can solve the problems of unfriendly experts in the field of description methods, difficult formal verification, and lack of readability.
- Summary
- Abstract
- Description
- Claims
- Application Information
AI Technical Summary
Problems solved by technology
Method used
Image
Examples
Embodiment
[0079] The method of the present invention will be further described in combination with specific embodiments and accompanying drawings for modeling an automatic driving lane change and overtaking scene model based on the automatic driving scene modeling language ADSML.
[0080] refer to figure 1 , this embodiment uses the automatic driving scene modeling language ADSML to model the scene model of automatic driving lane change and overtaking:
[0081] S1: Spatio-temporal trajectory data collection: By collecting the static and dynamic environmental data during the driving process of the self-driving car in the real environment, the spatio-temporal trajectory data for scene modeling in the field of autonomous driving is formed, as follows:
[0082] S1-A: First use OSM to obtain the road network structure around the self-driving vehicle, use GPS positioning technology to obtain the real-time positioning of the self-driving vehicle to obtain its trajectory data, and detect all di...
PUM
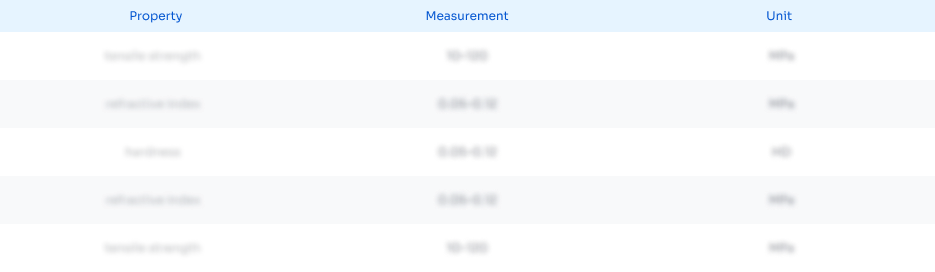
Abstract
Description
Claims
Application Information
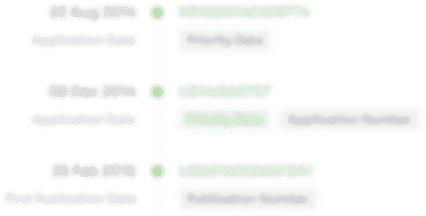
- R&D Engineer
- R&D Manager
- IP Professional
- Industry Leading Data Capabilities
- Powerful AI technology
- Patent DNA Extraction
Browse by: Latest US Patents, China's latest patents, Technical Efficacy Thesaurus, Application Domain, Technology Topic, Popular Technical Reports.
© 2024 PatSnap. All rights reserved.Legal|Privacy policy|Modern Slavery Act Transparency Statement|Sitemap|About US| Contact US: help@patsnap.com