Multilayer network clustering method based on semi-supervision
A multi-layer network and clustering method technology, applied in the field of multi-layer network clustering based on semi-supervised, can solve problems such as a lot of noise and sparse structure, and achieve the effect of effective mining and high accuracy
- Summary
- Abstract
- Description
- Claims
- Application Information
AI Technical Summary
Problems solved by technology
Method used
Image
Examples
Embodiment 1
[0087] The internal density function f(D) in the step S3 is defined as:
[0088]
[0089] Among them, m D Indicates the number of edges actually contained in all nodes in the dense subgraph D; n D (n D -1) / 2 represents the total number of edges owned by the dense subgraph D.
Embodiment 2
[0091] In the fusion optimization process of the consensus matrix H in step S6, the consensus prior matrix P is continuously used to minimize the dissimilarity of the row vectors corresponding to the nodes in H that have a high probability of belonging to the same cluster, and these nodes will eventually be Divided into the same cluster, for node υ i and υ j The similarity of , measured using the square of the Euclidean distance, node υ i and υ j The corresponding row vector h of row i and row j in H i and h j The calculation method of the similarity between them is:
[0092]
[0093] Among them, h i and h j represent the row vectors of the i-th row and the j-th row in the consensus matrix H respectively; υ i and υ j The more similar, then S(h i ,h j ) the smaller the value.
Embodiment 3
[0095] The specific encoding method in the step S7 is as follows:
[0096]
[0097] where P is a binary diagonal matrix, p ij =1 means υ i and υ j The probability of being divided into the same cluster increases; H is the consensus low-dimensional representation matrix of the multi-layer network; S(h i ,h j ) is used to measure h i and h j the similarity of is a diagonal matrix whose diagonal entries are L=D-P is defined as the graph regularization matrix of P; Tr(·) denotes the trace of the matrix.
[0098] refer to figure 2 , shows a detailed diagram of the method framework of the present invention. Specifically, the method (S-MCGR) proposed by the present invention mainly includes three core components. (1) Use the greedy search algorithm to obtain the consensus prior information of the multi-layer network and initialize each network layer; (2) Encode the obtained consensus prior information into the regularization term of the consensus subspace graph; (3) B...
PUM
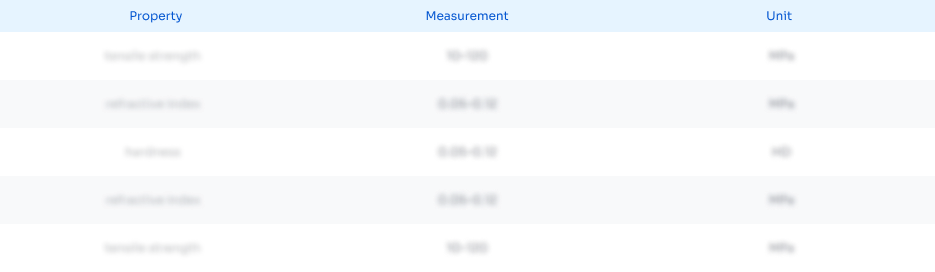
Abstract
Description
Claims
Application Information
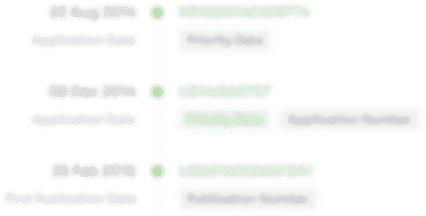
- R&D Engineer
- R&D Manager
- IP Professional
- Industry Leading Data Capabilities
- Powerful AI technology
- Patent DNA Extraction
Browse by: Latest US Patents, China's latest patents, Technical Efficacy Thesaurus, Application Domain, Technology Topic, Popular Technical Reports.
© 2024 PatSnap. All rights reserved.Legal|Privacy policy|Modern Slavery Act Transparency Statement|Sitemap|About US| Contact US: help@patsnap.com