Dense correspondence prediction method based on non-rigid point cloud
A prediction method, non-rigid technology, applied in the field of 3D reconstruction, can solve problems such as failure of deformation optimization
- Summary
- Abstract
- Description
- Claims
- Application Information
AI Technical Summary
Problems solved by technology
Method used
Image
Examples
Embodiment Construction
[0055] Such as figure 1 As shown, a dense corresponding prediction method based on non-rigid point cloud, using cascaded graph convolutional neural network and multiple set abstraction layers to extract geometric features of 3D template model and point cloud respectively; using global regression network according to template Infer the global displacement with the associated global feature of the point cloud; use the local feature embedding technology and introduce the attention mechanism to fuse the local depth feature of the point cloud with the geometric feature of the graph; use the local regression network to predict the displacement increment; use the weakly supervised A fine-tuning method, robust to real point clouds, and unified with a two-stage regression network within a complete framework. Specific steps are as follows:
[0056] Step 1. Use the cascaded Chebyshev spectral graph convolutional neural network to obtain the geometric feature F on the 3D template grid 1...
PUM
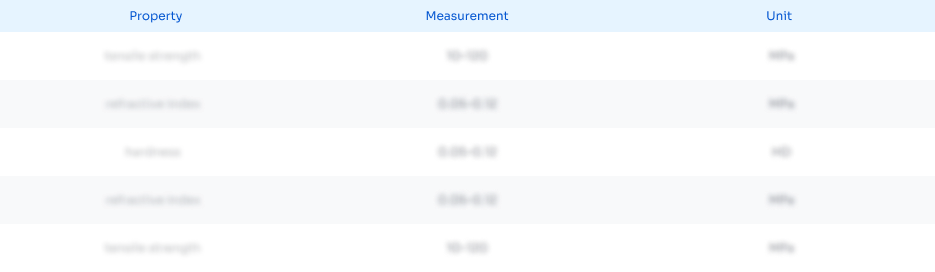
Abstract
Description
Claims
Application Information
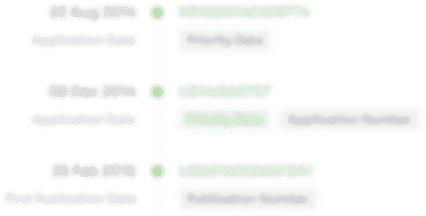
- Generate Ideas
- Intellectual Property
- Life Sciences
- Materials
- Tech Scout
- Unparalleled Data Quality
- Higher Quality Content
- 60% Fewer Hallucinations
Browse by: Latest US Patents, China's latest patents, Technical Efficacy Thesaurus, Application Domain, Technology Topic, Popular Technical Reports.
© 2025 PatSnap. All rights reserved.Legal|Privacy policy|Modern Slavery Act Transparency Statement|Sitemap|About US| Contact US: help@patsnap.com