Cross-domain image classification method based on pseudo label domain adaptation
A classification method and a technology for labeling domains, applied in neural learning methods, instruments, biological neural network models, etc., can solve the problems of expensive labeling data and difficulty in obtaining data
- Summary
- Abstract
- Description
- Claims
- Application Information
AI Technical Summary
Problems solved by technology
Method used
Image
Examples
Embodiment Construction
[0029] In order to make the object, technical solution and advantages of the present invention clearer, the following examples will further describe the implementation of the present invention in conjunction with the accompanying drawings.
[0030] To deal with the problem of poor generalization performance of the target domain on the model trained in the source domain, the present invention screens out a part of high-confidence samples from the target domain, uses the model prediction results as the pseudo-labels of the corresponding samples, and then adds the pseudo-label samples to the to the training set to train the network. Such alternate false labeling and network training can gradually learn the discriminative features of the target domain and further improve the generalization ability of the model in the target domain. The training process is as figure 1 , the present invention first performs pre-training on the source domain, and then enhances the generalization per...
PUM
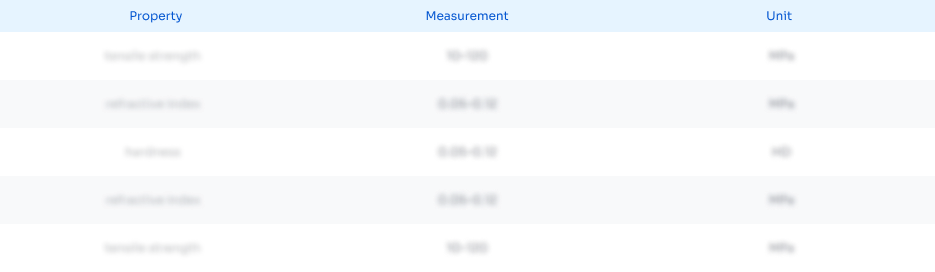
Abstract
Description
Claims
Application Information
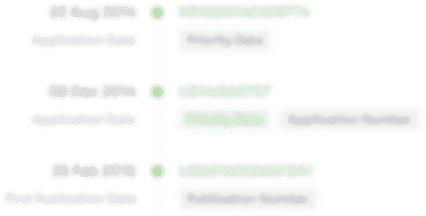
- R&D Engineer
- R&D Manager
- IP Professional
- Industry Leading Data Capabilities
- Powerful AI technology
- Patent DNA Extraction
Browse by: Latest US Patents, China's latest patents, Technical Efficacy Thesaurus, Application Domain, Technology Topic.
© 2024 PatSnap. All rights reserved.Legal|Privacy policy|Modern Slavery Act Transparency Statement|Sitemap