Self-adaptive signal analysis method based on continuous variable variational mode decomposition
A technique of variational mode decomposition and adaptive signal, which is applied in the field of analysis and processing of multivariate data, to achieve the effect of solving mode aliasing, less parameters and low computational complexity
- Summary
- Abstract
- Description
- Claims
- Application Information
AI Technical Summary
Problems solved by technology
Method used
Image
Examples
Embodiment Construction
[0043] Below in conjunction with embodiment, the content of the invention is further described.
[0044] First, use the simulation data to verify the effect of adaptive decomposition, denoising and anti-modal aliasing of the method of the present invention, and then apply the actual EEG signal to verify that the method of the present invention is applicable to the early prediction of epileptic seizures:
[0045] 1. Test the robustness of the algorithm to noise, and compare the decomposition effects of SMVMD and MEMD. Construct a composite cosine signal f with Gaussian white noise 1 and f 2 and the noise-free signal f 3 , whose composition is as follows:
[0046] f 1 =4cos(10πt)+2cos(20πt)+η 1 ,
[0047] f 2 =3cos(10πt)+3cos(50πt)+η 2 ,
[0048] f 3 =2cos(10πt)+3cos(20πt)+4cos(50πt),
[0049] where η 1 and η 2 Gaussian white noise, η 1 ~N(0,0.12), η 2 ~N(0,0.12), the signal waveform is shown in Figure 1(a). In both simulation experiments and real EEG data proces...
PUM
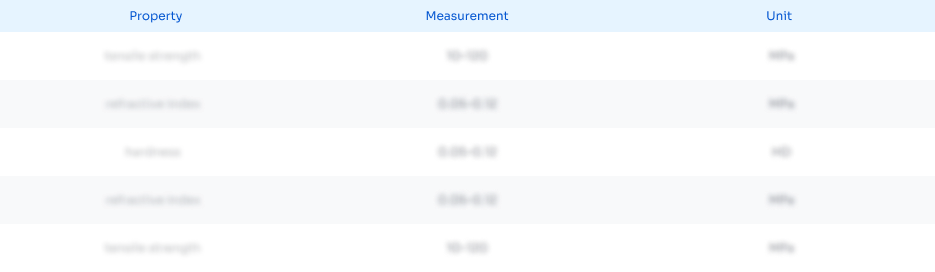
Abstract
Description
Claims
Application Information
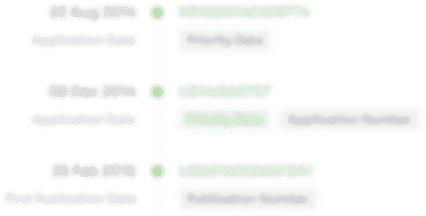
- R&D Engineer
- R&D Manager
- IP Professional
- Industry Leading Data Capabilities
- Powerful AI technology
- Patent DNA Extraction
Browse by: Latest US Patents, China's latest patents, Technical Efficacy Thesaurus, Application Domain, Technology Topic, Popular Technical Reports.
© 2024 PatSnap. All rights reserved.Legal|Privacy policy|Modern Slavery Act Transparency Statement|Sitemap|About US| Contact US: help@patsnap.com