Real-time cloth defect detection method and system based on deep learning
A defect detection and deep learning technology, applied in neural learning methods, image analysis, image enhancement and other directions, can solve the problems of unsatisfactory detection speed, insufficient robustness, and unsatisfactory effect, and achieves the elimination of manual design features, Improve the degree of intelligence and improve the effect of detection performance
- Summary
- Abstract
- Description
- Claims
- Application Information
AI Technical Summary
Problems solved by technology
Method used
Image
Examples
Embodiment Construction
[0049] It should be noted that, in the case of no conflict, the embodiments in the present application and the features in the embodiments can be combined with each other. The present invention will be further described in detail below in conjunction with the drawings and specific embodiments.
[0050] The real-time cloth defect detection method based on deep learning in the embodiment of the present invention first uses the generative confrontation network to generate the required defect data, and then uses the deep learning target detection network for training, and saves it after training to obtain the optimal model. Load the model file into the network, input a cloth image at this time, and the real-time cloth defect detection system based on deep learning in the embodiment of the present invention can automatically infer the result through the model.
[0051] Please refer to Figure 1 ~ Figure 3 , the deep learning-based real-time cloth defect detection method of the embo...
PUM
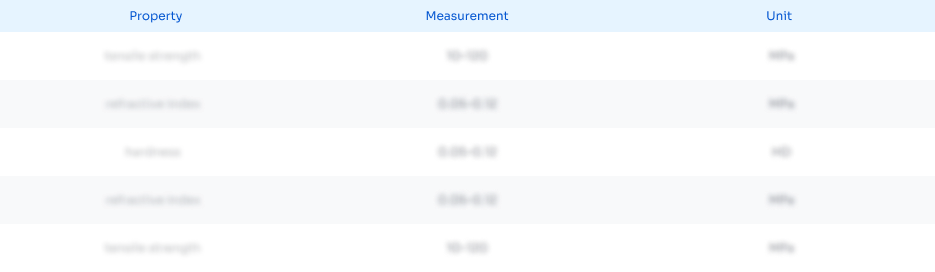
Abstract
Description
Claims
Application Information
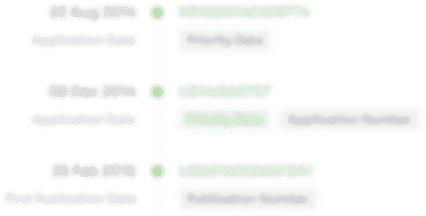
- R&D Engineer
- R&D Manager
- IP Professional
- Industry Leading Data Capabilities
- Powerful AI technology
- Patent DNA Extraction
Browse by: Latest US Patents, China's latest patents, Technical Efficacy Thesaurus, Application Domain, Technology Topic, Popular Technical Reports.
© 2024 PatSnap. All rights reserved.Legal|Privacy policy|Modern Slavery Act Transparency Statement|Sitemap|About US| Contact US: help@patsnap.com