Medical image deep learning method with interpretability
A medical image and deep learning technology, applied in the field of medical image deep learning, can solve problems such as blurred border pixels, missed or misdiagnosed lesion areas, and easy confusion of surrounding normal physiological features.
- Summary
- Abstract
- Description
- Claims
- Application Information
AI Technical Summary
Problems solved by technology
Method used
Image
Examples
example
[0093] The specific operation process of the present invention will be further described below by way of example. The examples use datasets from the 2018 and 2019 ISIC Glandular Cancer Detection Challenge.
[0094] In the data set used, the 2018 data set has a total of 2594 training images and 1000 test data images, with standard data images (groundtruth); the 2019 data set has a total of 2531 training data images, 823 test data images, also attached There are standard data images (groundtruth). In the training phase, the learning rate is set to 0.005, the number of samples (batch size) for one training is 2000, and the number of iterations is 100.
[0095] Define precision (Precision) and recall (Recall) as the evaluation indicators of the model, namely
[0096]
[0097]
[0098] In the formula, TP represents the number of True Positives, FP represents the number of False Positives, and FN represents the number of False Negatives.
PUM
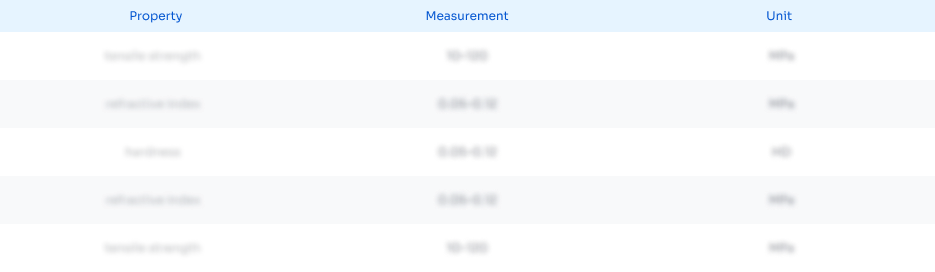
Abstract
Description
Claims
Application Information
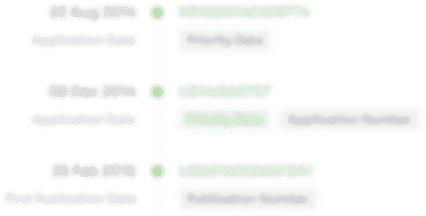
- R&D Engineer
- R&D Manager
- IP Professional
- Industry Leading Data Capabilities
- Powerful AI technology
- Patent DNA Extraction
Browse by: Latest US Patents, China's latest patents, Technical Efficacy Thesaurus, Application Domain, Technology Topic, Popular Technical Reports.
© 2024 PatSnap. All rights reserved.Legal|Privacy policy|Modern Slavery Act Transparency Statement|Sitemap|About US| Contact US: help@patsnap.com