Blurred image restoration method based on unsupervised generative adversarial network
A fuzzy image, unsupervised technology, applied in biological neural network models, image enhancement, image analysis, etc., can solve the problems of noise sensitivity, insufficient network generalization, limited training data sets, etc., to reduce workload and effectively The effect of practical value
- Summary
- Abstract
- Description
- Claims
- Application Information
AI Technical Summary
Problems solved by technology
Method used
Image
Examples
Embodiment Construction
[0039] The technical solution of the present invention will be further described in detail below in conjunction with the accompanying drawings and embodiments.
[0040] like figure 1 , a blurred image restoration method based on an unsupervised generation confrontation network of the present invention, comprising the following steps:
[0041] Step 1. Construct unsupervised datasets, which are image datasets in the fuzzy image domain and image datasets in the clear image domain, denoted as S 1 ,S 2 , and the data in the two sets are not in one-to-one correspondence.
[0042] Step 2, such as figure 2 As shown, under the framework of the generative confrontation network, two dual generation paths with reciprocal directions are set, which are: input fuzzy image-generate clear image-reconstruct fuzzy image (denoted as A direction), input clear image-generate fuzzy image - Reconstruct a clear image (denoted as B direction). Each generation path uses a generator network G A , ...
PUM
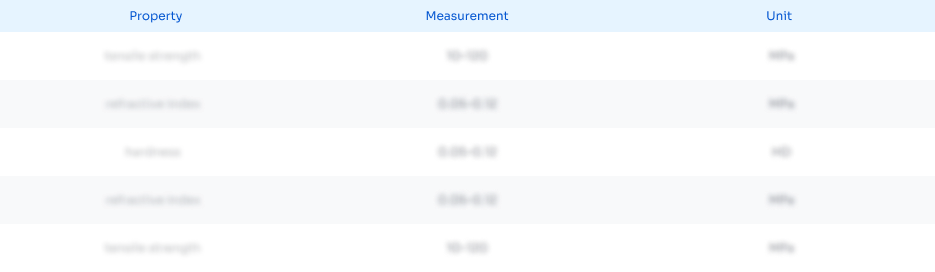
Abstract
Description
Claims
Application Information
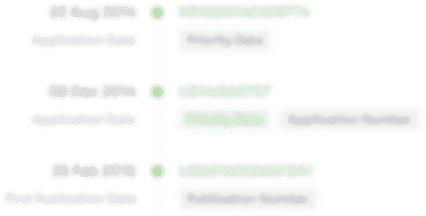
- R&D Engineer
- R&D Manager
- IP Professional
- Industry Leading Data Capabilities
- Powerful AI technology
- Patent DNA Extraction
Browse by: Latest US Patents, China's latest patents, Technical Efficacy Thesaurus, Application Domain, Technology Topic, Popular Technical Reports.
© 2024 PatSnap. All rights reserved.Legal|Privacy policy|Modern Slavery Act Transparency Statement|Sitemap|About US| Contact US: help@patsnap.com