A neural network-based fall detection method for multi-scale and multi-target
A detection method and neural network technology, applied in neural learning methods, biological neural network models, neural architectures, etc., can solve problems such as large impact, impact on detection speed, low accuracy, etc., to reduce training difficulty, improve detection speed, The effect of improving accuracy
- Summary
- Abstract
- Description
- Claims
- Application Information
AI Technical Summary
Problems solved by technology
Method used
Image
Examples
specific Embodiment approach 1
[0039] A neural network-based fall detection method applicable to multi-scale and multi-target in this embodiment, combined with figure 1 , the method includes the following steps:
[0040] Step 1. Supplement the public fall detection data set and make a fall detection fusion data set;
[0041] Step 2. Transform the YOLOv4 network to build a YOLOv4 fall detection network for human body characteristics;
[0042] Step 3. Use the K-means algorithm to update the anchors value for the fall detection fusion data set;
[0043] Step 4, use Label Smoothing to transform the network label;
[0044] Step 5: Train and test the transformed YOLOv4 network.
specific Embodiment approach 2
[0045] The difference from the specific embodiment 1 is that a neural network-based fall detection method suitable for multi-scale and multi-target in this embodiment, combined with figure 2 , the specific process of making a fall detection fusion data set in the step 1 is:
[0046] (1) Make fusion video: Shoot multiple human body targets at different distances from the camera at different angles, single-person and multi-person fall videos, and fuse them with public fall videos. The human body movements in the video include standing, walking, sitting, Bending and falling; shooting scenes include conference room, bedroom at home;
[0047] (2) Frame interception and preliminary screening: Frame interception is performed on the fused video, three frames per second are intercepted, and video data at different times are selected to prevent the impact caused by changes in light, background, and clothing. After preliminary screening, 13746 an original image;
[0048] (3) Further s...
specific Embodiment approach 3
[0051] The difference from the first or second specific embodiment is that a neural network-based fall detection method suitable for multi-scale and multi-target in this embodiment, combined with image 3 , the specific process of building a YOLOv4 fall detection network for human body characteristics in the second step is as follows:
[0052] (1) Build a YOLOv4 feature extraction network CSPDarknet53 for human characteristics: The YOLOv4 network model uses three feature layers (13, 13, N), (26, 26, N), (52, 52, N) respectively, which are used to Recognize three types of targets, large, medium and small. Due to the large size of the human body, in order to suit the N characteristics of the human body size, the above three types of feature layers are transformed into (6, 6, N), (12, 12, N), (24, 24, N ) for detection, adjust the size of the input image to (384, 384), and add a layer of Resblock_body (6, 6, 1024)x1 layer;
[0053] (2) Build the neck network of YOLOv4: PANet net...
PUM
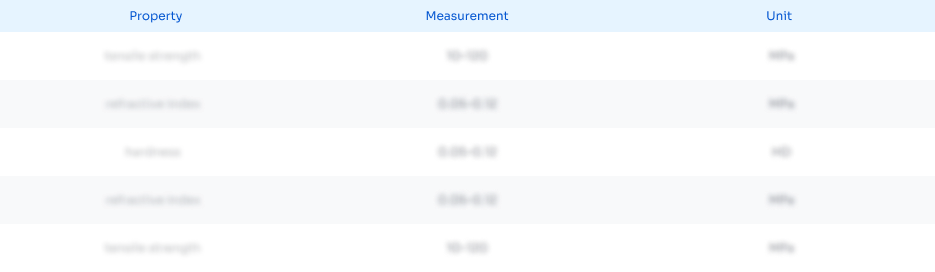
Abstract
Description
Claims
Application Information
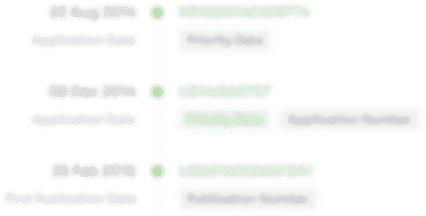
- R&D
- Intellectual Property
- Life Sciences
- Materials
- Tech Scout
- Unparalleled Data Quality
- Higher Quality Content
- 60% Fewer Hallucinations
Browse by: Latest US Patents, China's latest patents, Technical Efficacy Thesaurus, Application Domain, Technology Topic, Popular Technical Reports.
© 2025 PatSnap. All rights reserved.Legal|Privacy policy|Modern Slavery Act Transparency Statement|Sitemap|About US| Contact US: help@patsnap.com