Robust graph convolutional neural network method based on space-time sparse learning
A convolutional neural network, robust graph technology, applied in the field of graph adversarial attack and defense, can solve problems such as application crash and GNN vulnerable to adversarial attacks, achieve defense against adversarial attacks, improve robustness and stability Effect
- Summary
- Abstract
- Description
- Claims
- Application Information
AI Technical Summary
Problems solved by technology
Method used
Image
Examples
Embodiment Construction
[0028] The present invention provides an embodiment of a robust graph convolutional neural network method based on spatiotemporal sparse learning, in order to enable those skilled in the art to better understand the technical solutions in the embodiments of the present invention, and to make the above-mentioned purpose and characteristics of the present invention And advantage can be more obvious and easy to understand, below in conjunction with accompanying drawing technical scheme in the present invention is described in further detail:
[0029] Such as figure 1 As shown, a robust graph convolutional neural network method based on spatiotemporal sparse learning, based on the graph convolutional neural network, constructs robust feature representations through spatiotemporal sparse learning, not only can learn to activate the most salient features, and the potential set of active features can be expanded through temporal sparsification learning so that active features can be ...
PUM
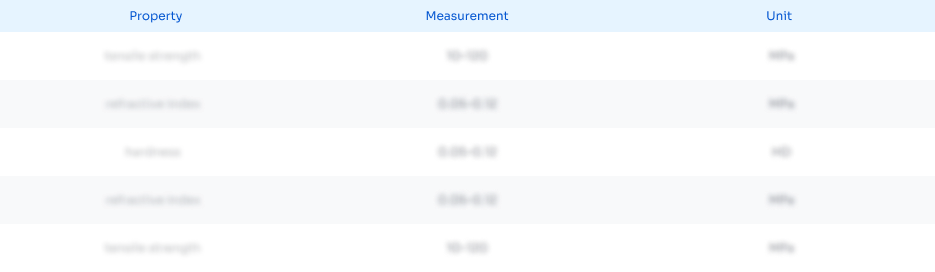
Abstract
Description
Claims
Application Information
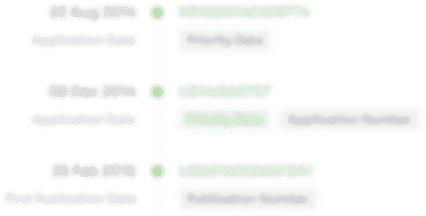
- R&D
- Intellectual Property
- Life Sciences
- Materials
- Tech Scout
- Unparalleled Data Quality
- Higher Quality Content
- 60% Fewer Hallucinations
Browse by: Latest US Patents, China's latest patents, Technical Efficacy Thesaurus, Application Domain, Technology Topic, Popular Technical Reports.
© 2025 PatSnap. All rights reserved.Legal|Privacy policy|Modern Slavery Act Transparency Statement|Sitemap|About US| Contact US: help@patsnap.com