A Design Method for Second-Order Helmholtz Resonators Based on Deep Learning
A technology of deep learning and design method, applied in the field of deep learning, it can solve the problems of difficult analysis and solution of physical properties, too many geometric parameters, and time-consuming, achieving the effect of low computational cost, strong versatility, and improved design efficiency.
- Summary
- Abstract
- Description
- Claims
- Application Information
AI Technical Summary
Problems solved by technology
Method used
Image
Examples
Embodiment Construction
[0115] The technical solution of the present invention will be further described in detail below in conjunction with the accompanying drawings.
[0116] Such as figure 1 As shown, the second-order Helmholtz resonator of the present invention includes: a first resonance cavity and a second resonance cavity, the first resonance cavity includes a first cylindrical cavity and a first open cylinder at the bottom thereof, and the second resonance cavity The cavity includes a second cylindrical cavity and a second open cylinder at the bottom, wherein the first open cylinder communicates with the flow channel; the second open cylinder communicates with the top of the first cylindrical cavity; the second-order Helmholm The geometric parameters of the magnetic resonator include: the cavity depth h of the first cylindrical cavity 1 , the radius a of the first open cylinder 1 and length l 1 ;Cavity depth h of the second cylindrical cavity 2 , and the radius a of the second open cylind...
PUM
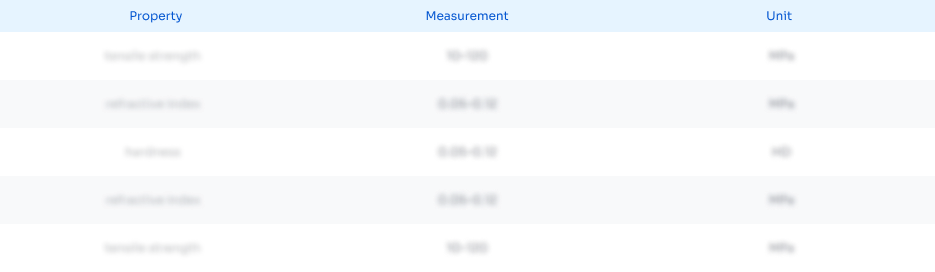
Abstract
Description
Claims
Application Information
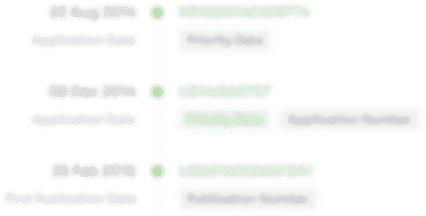
- R&D Engineer
- R&D Manager
- IP Professional
- Industry Leading Data Capabilities
- Powerful AI technology
- Patent DNA Extraction
Browse by: Latest US Patents, China's latest patents, Technical Efficacy Thesaurus, Application Domain, Technology Topic, Popular Technical Reports.
© 2024 PatSnap. All rights reserved.Legal|Privacy policy|Modern Slavery Act Transparency Statement|Sitemap|About US| Contact US: help@patsnap.com