Lung cancer prognosis auxiliary evaluation method and system based on CT radiomics
A radiomics, CT imaging technology, applied in computer-aided medical procedures, informatics, medical images, etc., to achieve individualized and precise medical treatment, and to solve the effect of insensitivity to radiotherapy efficacy
- Summary
- Abstract
- Description
- Claims
- Application Information
AI Technical Summary
Problems solved by technology
Method used
Image
Examples
Embodiment Construction
[0024] In order to make the purpose, technical solutions and advantages of the embodiments of the present invention clearer, the technical solutions in the embodiments of the present invention will be clearly and completely described below in conjunction with the drawings in the embodiments of the present invention. Obviously, the described embodiments It is a part of the embodiments of the present invention, rather than all embodiments; based on the embodiments of the present invention, all other embodiments obtained by those of ordinary skill in the art without creative work, all belong to the protection scope of the present invention .
[0025] Such as figure 1 As shown, an auxiliary assessment method for lung cancer prognosis based on CT radiomics, including: S101, collecting and screening the original medical images and clinical information of lung cancer patients treated with radiotherapy; S102, randomly dividing lung cancer patients into training set and verification s...
PUM
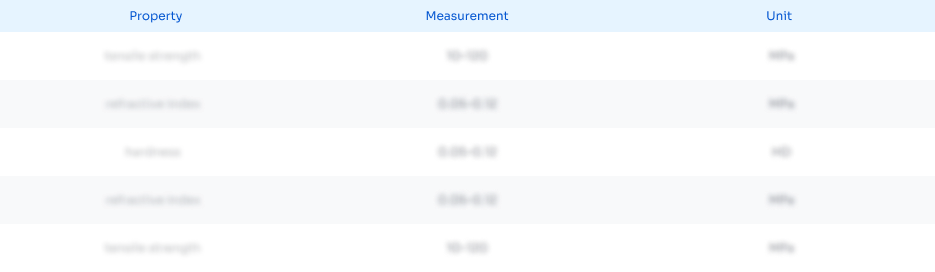
Abstract
Description
Claims
Application Information
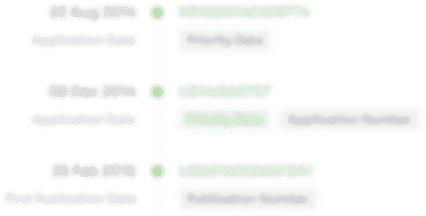
- Generate Ideas
- Intellectual Property
- Life Sciences
- Materials
- Tech Scout
- Unparalleled Data Quality
- Higher Quality Content
- 60% Fewer Hallucinations
Browse by: Latest US Patents, China's latest patents, Technical Efficacy Thesaurus, Application Domain, Technology Topic, Popular Technical Reports.
© 2025 PatSnap. All rights reserved.Legal|Privacy policy|Modern Slavery Act Transparency Statement|Sitemap|About US| Contact US: help@patsnap.com