Question and answer matching method based on adaptive fusion multi-attention network
A matching method and attention technology, applied in neural learning methods, biological neural network models, character and pattern recognition, etc., to achieve the effect of improving network performance
- Summary
- Abstract
- Description
- Claims
- Application Information
AI Technical Summary
Problems solved by technology
Method used
Image
Examples
Embodiment 1
[0053] Such as figure 1 As shown, a question and answer matching method based on adaptive fusion multi-attention network, including the following steps:
[0054] S1: Convert each word in the question and answer into a word vector, and encode the word vector in the question and answer to obtain the word vector sequence of the question and answer;
[0055] S2: Use multiple self-attention networks to extract different aspects of information in a question according to the sequence of word vectors, and encode them into different question vectors; among them, a self-attention network extracts information in one aspect of a question, corresponding to a question vector ;
[0056] S3: Use a sequential attention network to generate a corresponding answer vector for each question vector;
[0057] S4: Calculate the matching score of each question vector and its corresponding answer vector, and adaptively fuse the matching score of multiple aspects of information by evaluating the weight...
Embodiment 2
[0059] More specifically, such as figure 2 As shown, in step S1, a bidirectional LSTM is used to encode the word vectors in the question and the answer respectively, so as to obtain a word vector representation that includes context information.
[0060] More specifically, given a sentence S=(w 1 ,w 2 ,...,w l ), use the bidirectional LSTM to encode the word vector to obtain the corresponding hidden layer:
[0061]
[0062]
[0063]
[0064] When the given sentence S is a question, the hidden layer vector sequence H of each word vector in the question is obtained q ={h q (1),...,h q (l)}, put H q ={h q (1),...,h q (l)} as the word vector sequence of the question;
[0065] When the given sentence S is the answer, the hidden layer vector sequence H of each word vector in the answer is obtained a ={h a (1),...,h a (l)}, put H a ={h a (1),...,h a (l)} as the word vector sequence of the answer;
[0066] Among them, w 1 ,w 2 ,...,w l are the l words in t...
Embodiment 3
[0096] In this embodiment, three public data sets cited by many papers are selected as the evaluation data sets, and the empirical research of the question and answer matching method based on adaptive fusion multi-attention network is carried out.
[0097] Table 1 is the situation of three public datasets.
[0098] Table 1
[0099] data set TrecQA WikiQA InsuranceQA Number of train / validation / test questions 1162 / 65 / 68 873 / 126 / 243 12887 / 1000 / 1800x2 average question length 8 6 7 average answer length 28 25 95 Average number of candidate answers 38 9 500
[0100] In order to make a fair comparison with previous studies, this example continues to use the previous evaluation criteria on the same data set. On TrecQA and WikiQA, this example uses MeanAveragePrecision (MAP) and MeanReciprocalRank (MRR) to evaluate the performance of the model. On the other hand, InsuranceQA follows the top1 correct rate used by predecessors, and it...
PUM
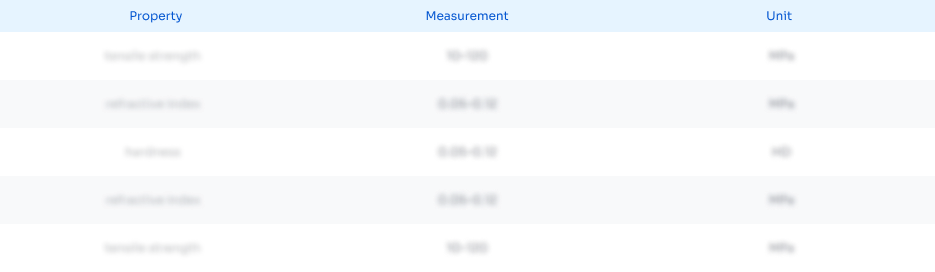
Abstract
Description
Claims
Application Information
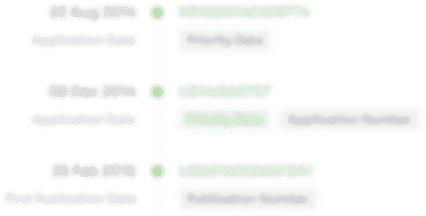
- R&D
- Intellectual Property
- Life Sciences
- Materials
- Tech Scout
- Unparalleled Data Quality
- Higher Quality Content
- 60% Fewer Hallucinations
Browse by: Latest US Patents, China's latest patents, Technical Efficacy Thesaurus, Application Domain, Technology Topic, Popular Technical Reports.
© 2025 PatSnap. All rights reserved.Legal|Privacy policy|Modern Slavery Act Transparency Statement|Sitemap|About US| Contact US: help@patsnap.com