Marketing activity prediction model structure and prediction method based on knowledge distillation
An activity prediction and knowledge technology, applied in the field of artificial intelligence in Internet marketing, can solve problems such as increased computing overhead, slow model response speed, delay, and computing resource limitations, to save marketing costs, improve accuracy, and achieve profit margins. Effect
- Summary
- Abstract
- Description
- Claims
- Application Information
AI Technical Summary
Problems solved by technology
Method used
Image
Examples
Embodiment Construction
[0065] The specific embodiment of the present invention will be further described in detail below in conjunction with the accompanying drawings.
[0066] In the following specific embodiments, when describing the embodiments of the present invention in detail, in order to clearly show the structure of the present invention for the convenience of description, the structures in the drawings are not drawn according to the general scale, and are partially enlarged and deformed. and simplified processing, therefore, it should be avoided to be interpreted as a limitation of the present invention.
[0067] see figure 1 , figure 1 Shown is a schematic flowchart of a marketing activity prediction method based on knowledge distillation in an embodiment of the present invention. Such as figure 1 As shown, the marketing activity prediction method based on knowledge distillation includes data preprocessing step S1, step S2 of data set division of teacher model and formation of network t...
PUM
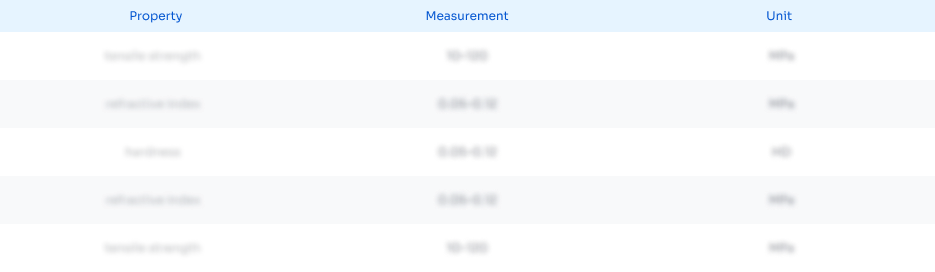
Abstract
Description
Claims
Application Information
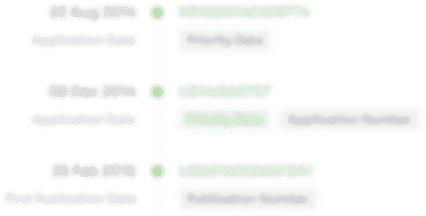
- R&D Engineer
- R&D Manager
- IP Professional
- Industry Leading Data Capabilities
- Powerful AI technology
- Patent DNA Extraction
Browse by: Latest US Patents, China's latest patents, Technical Efficacy Thesaurus, Application Domain, Technology Topic, Popular Technical Reports.
© 2024 PatSnap. All rights reserved.Legal|Privacy policy|Modern Slavery Act Transparency Statement|Sitemap|About US| Contact US: help@patsnap.com