Image compressed sensing reconstruction method based on residual dense threshold network
An image compression and dense technology, applied in image coding, image data processing, neural learning methods, etc., can solve problems such as the reconstruction quality needs to be improved, the reconstruction speed is slow, and the interpretability is poor
- Summary
- Abstract
- Description
- Claims
- Application Information
AI Technical Summary
Problems solved by technology
Method used
Image
Examples
Embodiment 1
[0050] Such as figure 1 As shown, this embodiment provides a method for image compression sensing reconstruction based on residual dense threshold network, including the following steps:
[0051] S1: The original image set is randomly cropped and divided into a series of original real cropped image blocks (high-dimensional original signal), and for each given cropped image block, it is converted into a one-dimensional vector, and at a selected sampling rate Construct the corresponding measurement matrix sampling through the random Gaussian matrix to obtain the corresponding sampling amount, and obtain a one-to-one corresponding training set;
[0052] In this embodiment, 91 original image sets are randomly cropped and segmented to obtain 88912 cropped image blocks with a size of 33×33. For each given cropped image block {x i |i=1,2,3,...,n}, and convert it into a one-dimensional vector, and construct the corresponding measurement matrix Φ from the generated random Gaussian mat...
Embodiment 2
[0071] This embodiment provides an image compression sensing reconstruction system based on a residual dense threshold network, including: a training set construction module, an initial reconstruction module, an optimized reconstruction module, an iterative training module, and a compressed sensing reconstruction module;
[0072] In this embodiment, the training set construction module is used to divide the original natural image into blocks and then sample through the Gaussian sampling matrix, and make the low-dimensional sampling amount and cropped image blocks into a training set;
[0073] In this embodiment, the initial reconstruction module is used to restore the low-dimensional sampling amount into a high-dimensional initial reconstruction image block through linear convolution;
[0074] In this embodiment, the optimized reconstruction module is used to optimize and reconstruct the initial reconstructed image through the built residual dense threshold network. The residua...
Embodiment 3
[0078] This embodiment provides a storage medium, the storage medium can be a storage medium such as ROM, RAM, magnetic disk, optical disk, etc., and the storage medium stores one or more programs. Residual Dense Thresholding Networks for Image Compressive Sensing Reconstruction.
PUM
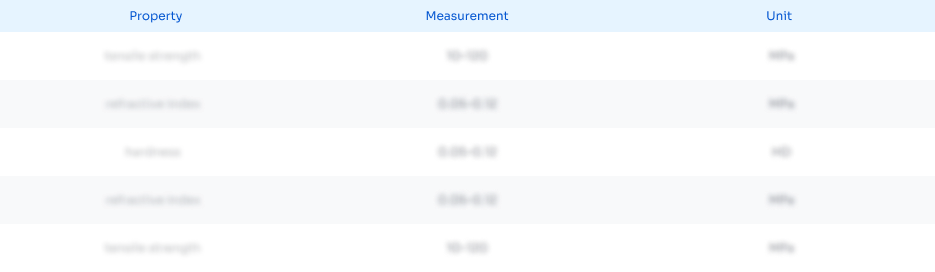
Abstract
Description
Claims
Application Information
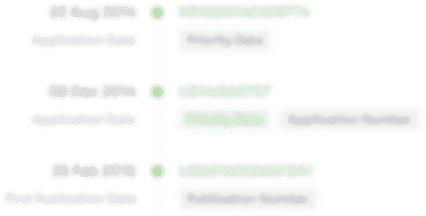
- Generate Ideas
- Intellectual Property
- Life Sciences
- Materials
- Tech Scout
- Unparalleled Data Quality
- Higher Quality Content
- 60% Fewer Hallucinations
Browse by: Latest US Patents, China's latest patents, Technical Efficacy Thesaurus, Application Domain, Technology Topic, Popular Technical Reports.
© 2025 PatSnap. All rights reserved.Legal|Privacy policy|Modern Slavery Act Transparency Statement|Sitemap|About US| Contact US: help@patsnap.com