Retinal vessel segmentation method and device based on multi-scale attention network, and storage medium
A retinal blood vessel, attention technology, applied in neural learning methods, biological neural network models, image analysis, etc., can solve the problem of ignoring specific areas of scale changes
- Summary
- Abstract
- Description
- Claims
- Application Information
AI Technical Summary
Problems solved by technology
Method used
Image
Examples
Embodiment 1
[0070] A retinal vessel segmentation method based on Multi-Scale AttentionNet, such as figure 1 shown, including the following steps:
[0071] Step 1: Get the dataset;
[0072] Step 2: Dataset preprocessing;
[0073] Perform grayscale processing, adaptive histogram equalization, and gamma correction on the data set obtained in step 1;
[0074] Step 3: Construct a retinal vessel segmentation model;
[0075] Step 4: Training the retinal vessel segmentation model; refers to: use the PyTorch framework to train the retinal vessel segmentation model, the loss function uses the combination of the cross entropy loss function and the dice loss function to solve the class balance problem, the coefficient is 0.5, and the learning rate is set is 0.0002 and the number of iterations is 150.
[0076] Step 5: retinal vessel segmentation test;
[0077] Preprocessing the fundus retinal image test set (sequentially performing grayscale processing, adaptive histogram equalization, and gamma ...
Embodiment 2
[0080] According to a kind of retinal blood vessel segmentation method based on multi-scale attention network (Multi-ScaleAttentionNet) described in embodiment 1, its difference is:
[0081] In step 2, data set preprocessing includes the following steps:
[0082] Step 2.1: Perform grayscale processing on the data set obtained in step 1, and convert all pictures into grayscale images; figure 2 It is a schematic diagram of the retinal grayscale image of tra_40, one of the pictures in the training set in the existing DRIVE dataset.
[0083] Step 2.2: Perform contrast-limited adaptive histogram equalization on the grayscale image obtained in step 2.1; the contrast-limited adaptive histogram equalization algorithm can effectively limit noise amplification.
[0084] Step 2.3: Use gamma correction to perform non-linear operations on the image obtained after processing in step 2.2. This will reduce the noise of the image and improve the overall contrast of blood vessels, which is b...
Embodiment 3
[0090] According to a kind of retinal blood vessel segmentation method based on multi-scale attention network (Multi-ScaleAttentionNet) described in embodiment 2, its difference is:
[0091] Such as Figure 5 As shown, the retinal vessel segmentation model includes a multi-scale connection network and an attention module;
[0092] The multi-scale connection network includes an encoding path and a decoding path. Both the encoding path and the decoding path include four spatially scaled blocks, and each spatially scaled block is passed through twice a 2D convolutional filter of size 3×3, ReLU, and batch normalization. (BN) layer, and the input and the output feature map of the input through the convolutional layer are connected; this will reduce overfitting and reduce the size of the input by half, which is beneficial for the network to learn contextual information.
[0093] In the encoding path, in each spatial scale block, the input and the output feature map of the input thr...
PUM
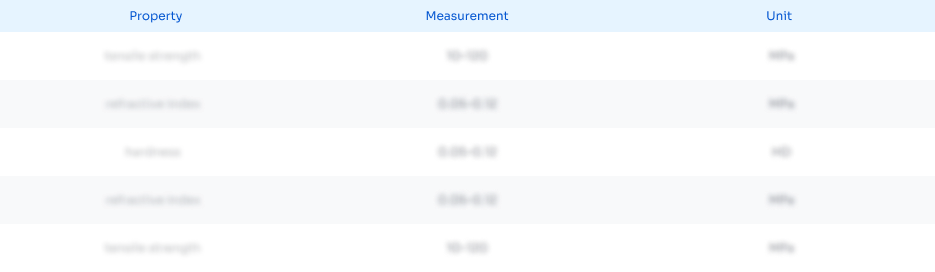
Abstract
Description
Claims
Application Information
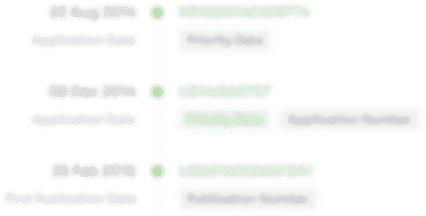
- R&D Engineer
- R&D Manager
- IP Professional
- Industry Leading Data Capabilities
- Powerful AI technology
- Patent DNA Extraction
Browse by: Latest US Patents, China's latest patents, Technical Efficacy Thesaurus, Application Domain, Technology Topic, Popular Technical Reports.
© 2024 PatSnap. All rights reserved.Legal|Privacy policy|Modern Slavery Act Transparency Statement|Sitemap|About US| Contact US: help@patsnap.com