Scene graph generation method based on super relation learning network
A technology for learning networks and super-relationships, applied in neural learning methods, biological neural network models, instruments, etc., it can solve the problems that no method considers high-level connections, and the performance impact is particularly significant, and achieves efficient distributed training. Significant, high inference and integration effects
- Summary
- Abstract
- Description
- Claims
- Application Information
AI Technical Summary
Problems solved by technology
Method used
Image
Examples
Embodiment Construction
[0098] The detailed parameters of the present invention will be further specifically described below.
[0099] Such as figure 1 , 2 , 3 and 4, the present invention provides a scene graph generation method (HLN) based on Hyper-relationship Learning Network (Hyper-relationship Learning Network).
[0100] The target frame generation network described in step (1) is as follows:
[0101] 1-1. The backbone network adopts the method of ResNetXt-101-FPN. The feature dimension extracted by the backbone network is 256. And the effect of data enhancement is not used, that is, the way of image flipping is not used.
[0102] 1-2. The candidate frame size of the Region Proposal Network is selected from (32,64,128,256,512). Each layer of FPN in training and testing selects 1000 candidate boxes, a total of 4 layers. The feature dimension of the frame extraction after the region generation network is 256.
[0103] 1-3. During the sampling process of the target frame, 64 target frames a...
PUM
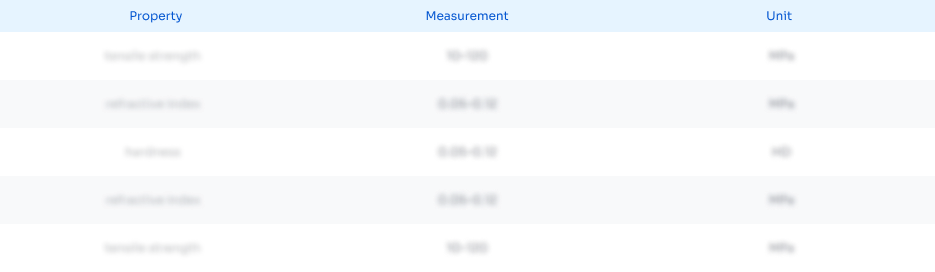
Abstract
Description
Claims
Application Information
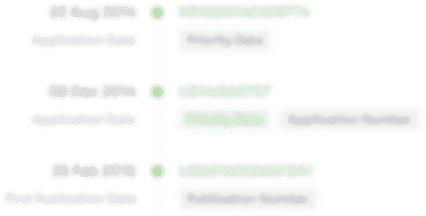
- R&D
- Intellectual Property
- Life Sciences
- Materials
- Tech Scout
- Unparalleled Data Quality
- Higher Quality Content
- 60% Fewer Hallucinations
Browse by: Latest US Patents, China's latest patents, Technical Efficacy Thesaurus, Application Domain, Technology Topic, Popular Technical Reports.
© 2025 PatSnap. All rights reserved.Legal|Privacy policy|Modern Slavery Act Transparency Statement|Sitemap|About US| Contact US: help@patsnap.com