Multi-sensor deep fusion 3D target detection method for automatic driving
A multi-sensor, autonomous driving technology, applied in the field of machine vision, can solve the problems of performance limitations, fusion network performance limitations, loss of information, etc., to improve robustness and accuracy, reduce the interference between multimodal information, Enhance the effect of complementarity
- Summary
- Abstract
- Description
- Claims
- Application Information
AI Technical Summary
Problems solved by technology
Method used
Image
Examples
Embodiment Construction
[0020] The technical solutions in the embodiments of the present invention will be clearly and completely described below in conjunction with the accompanying drawings in the embodiments of the present invention. Obviously, the described embodiments are only some of the embodiments of the present invention, not all of them. Based on the embodiments of the present invention, all other embodiments obtained by persons of ordinary skill in the art without making creative efforts belong to the protection scope of the present invention.
[0021] Multi-modal fusion is a future direction to improve perception accuracy and robustness. However, problems such as multi-sensor spatiotemporal asynchrony, inconsistent feature spaces of different modalities, and information loss in conversion make multi-modal fusion in autonomous driving scene perception difficult. The results do not exceed the best results for a single modality. In view of the above problems, the present invention provides a...
PUM
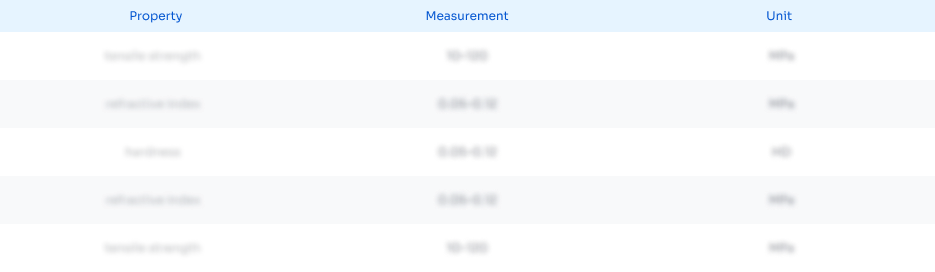
Abstract
Description
Claims
Application Information
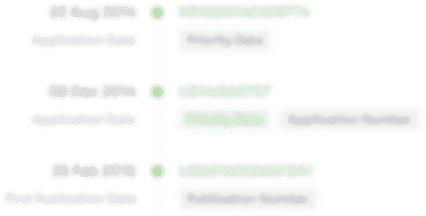
- R&D Engineer
- R&D Manager
- IP Professional
- Industry Leading Data Capabilities
- Powerful AI technology
- Patent DNA Extraction
Browse by: Latest US Patents, China's latest patents, Technical Efficacy Thesaurus, Application Domain, Technology Topic, Popular Technical Reports.
© 2024 PatSnap. All rights reserved.Legal|Privacy policy|Modern Slavery Act Transparency Statement|Sitemap|About US| Contact US: help@patsnap.com