Image restoration method and system based on equality structure multiple regularization
An image restoration and equation technology, applied in the direction based on specific mathematical models, image enhancement, image data processing, etc., can solve the problem that the multiple regularization BCS method has not yet appeared and cannot be used, and achieves avoiding covariance matrix and fast convergence speed. , the effect of reducing memory requirements and computational effort
- Summary
- Abstract
- Description
- Claims
- Application Information
AI Technical Summary
Problems solved by technology
Method used
Image
Examples
Embodiment 1
[0060] Such as figure 1 As shown, the image restoration method based on multiple regularization of the equation structure provided by this embodiment introduces multiple sparse transformations through the equation structure, that is, introduces multiple independent Delta probability density functions, and establishes multiple The restricted multi-level Bayesian model gives sparsity assumptions for the transformation coefficients corresponding to each transformation, so as to establish the conjugate matching relationship between each level, and finally combine the conjugate gradient method with the Expectation maximization (EM) method (conjugate gradient method, CGM) iteratively achieves fast reconstruction of the target image.
[0061] An image restoration method based on multiple regularization of an equation structure, comprising the following steps:
[0062] Step (1): On the premise of knowing the measurement matrix and observation vector, establish a linear equation for i...
Embodiment 2
[0171] This embodiment provides an image restoration system based on multiple regularization of an equation structure, including:
[0172] An image receiving module, which is used to receive the image to be restored, convert the image into a one-dimensional signal, and project the measurement matrix to the observation vector;
[0173] An image restoration module, which is used to input the measurement matrix and observation vector into the image restoration model based on multiple regularization of the equation structure to obtain the mean value of the target image; wherein, the image restoration model uses the conjugate gradient method to calculate the target value according to the measurement matrix and the observation vector Image mean and intermediate variable; use the calculated intermediate variable and target image mean to update the hyperparameter vector and equation variance of Bayesian compressive sensing; judge whether the convergence condition is satisfied, and if s...
Embodiment 3
[0175] This embodiment also provides an electronic device, including a memory, a processor, and computer instructions stored in the memory and run on the processor. When the computer instructions are executed by the processor, the steps of the method described in Embodiment 1 are completed. .
PUM
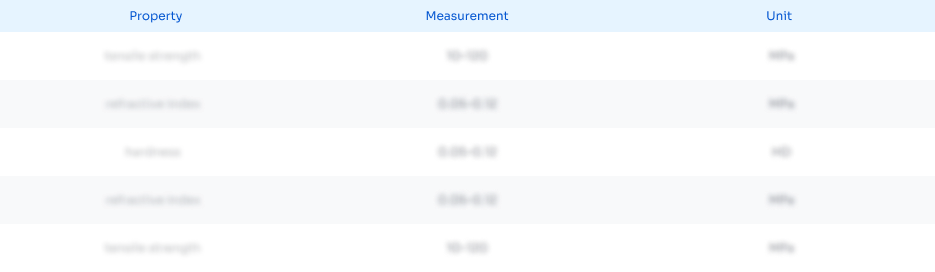
Abstract
Description
Claims
Application Information
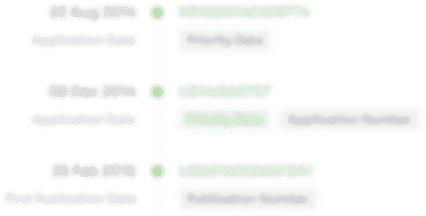
- R&D
- Intellectual Property
- Life Sciences
- Materials
- Tech Scout
- Unparalleled Data Quality
- Higher Quality Content
- 60% Fewer Hallucinations
Browse by: Latest US Patents, China's latest patents, Technical Efficacy Thesaurus, Application Domain, Technology Topic, Popular Technical Reports.
© 2025 PatSnap. All rights reserved.Legal|Privacy policy|Modern Slavery Act Transparency Statement|Sitemap|About US| Contact US: help@patsnap.com