Rolling bearing fault diagnosis method based on multi-scale convolutional neural network
A convolutional neural network and rolling bearing technology, applied in the field of intelligent fault diagnosis, can solve problems such as difficulty in being satisfied, and achieve the effect of reducing influence and dependence
- Summary
- Abstract
- Description
- Claims
- Application Information
AI Technical Summary
Problems solved by technology
Method used
Image
Examples
Embodiment Construction
[0037] The specific embodiments of the present invention will be described below in conjunction with the accompanying drawings.
[0038] Step 1: Decompose the original vibration signal into eigenmode components by adaptive variational mode decomposition, the specific steps are as follows:
[0039] Step 11: Obtain the original vibration signal: use the sensor to measure the original vibration signal,
[0040] Step 12: Let the number of initialized modal components K=2;
[0041] Step 13: The original vibration signal is iteratively calculated by the alternating multiplier method until convergence:
[0042]
[0043]
[0044] in is the original vibration signal; is the kth eigenmode component decomposed after n iterations; is the ith eigenmode component before the nth iteration; is the Lagrangian multiplier, which can be a constant 0; and are the k-th center frequency after the nth iteration and before the iteration respectively; ω is the angular frequency;
[...
PUM
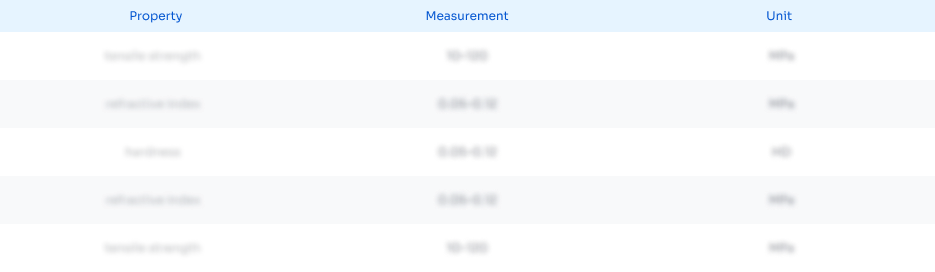
Abstract
Description
Claims
Application Information
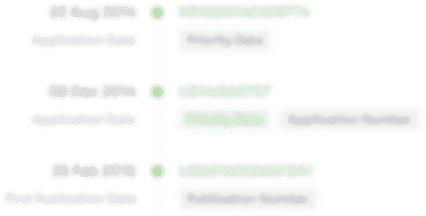
- R&D
- Intellectual Property
- Life Sciences
- Materials
- Tech Scout
- Unparalleled Data Quality
- Higher Quality Content
- 60% Fewer Hallucinations
Browse by: Latest US Patents, China's latest patents, Technical Efficacy Thesaurus, Application Domain, Technology Topic, Popular Technical Reports.
© 2025 PatSnap. All rights reserved.Legal|Privacy policy|Modern Slavery Act Transparency Statement|Sitemap|About US| Contact US: help@patsnap.com