Optimal neighbor multi-kernel clustering method and system based on local kernel
A kernel clustering and neighbor technology, applied in the field of data analysis, can solve the problems of low reliability, neglect, and insufficient consideration of the local density representation ability of a single data sample, and achieve the effect of excellent performance and improved performance.
- Summary
- Abstract
- Description
- Claims
- Application Information
AI Technical Summary
Problems solved by technology
Method used
Image
Examples
Embodiment 1
[0074] A local kernel-based optimal neighbor multi-kernel clustering method provided in this embodiment, such as figure 1 shown, including:
[0075] S11. Obtain clustering tasks and target data samples;
[0076] S12. Calculate the kernel matrix of each view corresponding to the target data sample, and perform centralization and normalization processing on the kernel matrix to obtain the processed kernel matrix;
[0077] S13. According to the obtained processed kernel matrix, establish an optimal neighbor multi-kernel clustering objective function based on the local kernel;
[0078] S14. Solving the established objective function in a cyclic manner to obtain a partition matrix after view fusion;
[0079] S15. Perform k-means clustering on the obtained partition matrix to obtain a clustering result.
[0080] This embodiment proposes a new method based on local kernel-based optimal neighbor multi-kernel clustering. Compared with existing methods, it includes building an adapti...
Embodiment 2
[0150] A local kernel-based optimal neighbor multi-core clustering method provided in this embodiment is different from Embodiment 1 in that:
[0151] The main content of this embodiment includes the design of an adaptive local kernel for the current multi-kernel clustering algorithm that does not fully consider the local density of a single data sample and the representation ability of the optimal kernel learned by over-restriction, and from the pre-specified The optimal kernel is located in the neighborhood of the linear combination of kernels; the two techniques are utilized in a single multi-kernel cluster framework; the generalization range of the optimal neighborhood multi-kernel clustering algorithm based on adaptive local kernels is studied.
[0152] The above-mentioned adaptive local kernel is a sub-matrix of the kernel function, and its main function is to reflect the relationship between the sample and its neighbors. First, define the threshold ζ, and the correspond...
Embodiment 3
[0169] A local kernel-based optimal neighbor multi-core clustering method provided in this embodiment is different from Embodiment 1 in that:
[0170] This embodiment is compared with existing methods on multiple data sets to verify the effectiveness of the present invention.
[0171] data set:
[0172] Flower102: This dataset contains 8189 samples evenly distributed in 102 categories with 4 kernel matrices.
[0173] Digital: This dataset includes 2000 samples, evenly distributed in 10 categories, with 3 kernel matrices.
[0174] Caltech101: This dataset contains 1530 samples uniformly distributed in 102 categories with 25 kernel matrices.
[0175] Protein Fold: This dataset includes 694 samples, evenly distributed in 27 categories, with 12 kernel matrices.
[0176] The statistics of the above datasets are shown in Table 2.
[0177]
[0178] Table 2
[0179] Data preparation and parameter setting:
[0180] In the initialization phase, follow [C.Cortes, M.Mohri, and A....
PUM
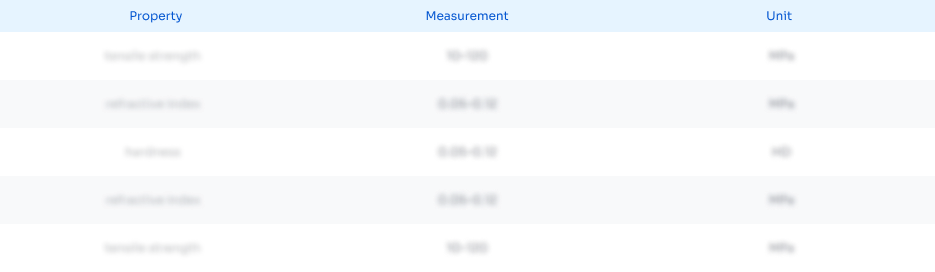
Abstract
Description
Claims
Application Information
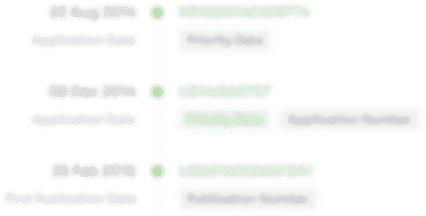
- R&D Engineer
- R&D Manager
- IP Professional
- Industry Leading Data Capabilities
- Powerful AI technology
- Patent DNA Extraction
Browse by: Latest US Patents, China's latest patents, Technical Efficacy Thesaurus, Application Domain, Technology Topic, Popular Technical Reports.
© 2024 PatSnap. All rights reserved.Legal|Privacy policy|Modern Slavery Act Transparency Statement|Sitemap|About US| Contact US: help@patsnap.com