An abductive inference approach to cross-focus loss based on pre-trained models
A reasoning method and pre-training technology, applied in the field of artificial intelligence, can solve problems such as assignment, low probability of occurrence, and no way to judge, and achieve the effect of improving robustness and accuracy.
- Summary
- Abstract
- Description
- Claims
- Application Information
AI Technical Summary
Problems solved by technology
Method used
Image
Examples
Embodiment 1
[0054] Such as figure 1 Shown, O 1 and O 2 For any observation pair, there are four corresponding hypotheses in this observation pair, where H 1 and H 2 For the correct assumption, H 3 and H 4 wrong assumption. The L2R2 ranking algorithm will rank these four probabilities and select the part with a higher probability as the correct answer. But there are still some flaws in it. First, for some hypotheses, we have no way to judge which one is more likely to be the answer, for example figure 1 H in 1 with H 2 , the meanings of these two sentences are not much different in essence, so it is difficult to compare their probabilities as answers. And for two unrelated answers, it is even more difficult to compare their probabilities as answers. Second, the L2R2 method sums the probabilities of all correct answers to a fixed value, when assuming H 1 When the probability of being the answer increases, the sum of the probabilities of other hypotheses will also decrease, which ...
Embodiment 2
[0093] According to the abductive inference method based on the cross focus loss of the pre-trained model described in embodiment 1, a certain event relationship classification based on clue mining is applied, and the specific facts are as follows:
[0094] Event relationship detection is a natural language processing technology that deeply determines the correlation between two events and what kind of logical relationship they have. Its core task is to use the event as the basic semantic unit, and realize the identification and judgment of the logical relationship between events by analyzing the semantic correlation features between relationship type) two research tasks. Event relationship recognition is mainly to judge whether there is a logical or semantic relationship between two events, and pre-collecting samples for deep relationship detection between events is an important prerequisite for in-depth analysis of event logic relationships; event relationship judgment is in...
PUM
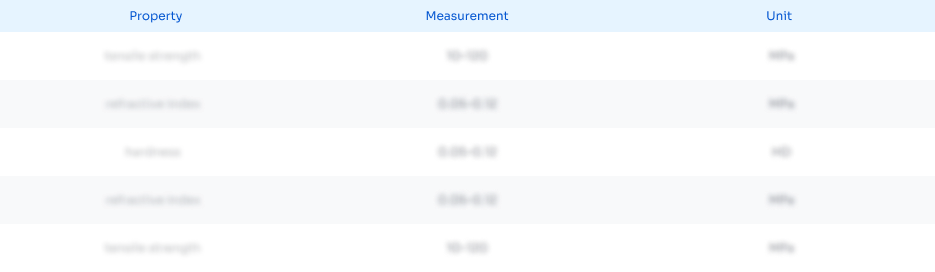
Abstract
Description
Claims
Application Information
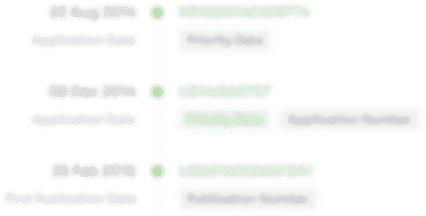
- R&D Engineer
- R&D Manager
- IP Professional
- Industry Leading Data Capabilities
- Powerful AI technology
- Patent DNA Extraction
Browse by: Latest US Patents, China's latest patents, Technical Efficacy Thesaurus, Application Domain, Technology Topic, Popular Technical Reports.
© 2024 PatSnap. All rights reserved.Legal|Privacy policy|Modern Slavery Act Transparency Statement|Sitemap|About US| Contact US: help@patsnap.com