Cell image segmentation method based on deep learning
An image segmentation and deep learning technology, applied in the field of image processing, can solve the problems of insufficient precision, high complexity of cell images, and low accuracy.
- Summary
- Abstract
- Description
- Claims
- Application Information
AI Technical Summary
Problems solved by technology
Method used
Image
Examples
Embodiment
[0039] In this embodiment, the specific steps of the cell image segmentation method based on deep learning are as follows:
[0040] (1) Acquire the image of the cell plate.
[0041] The image of the cell plate is obtained by taking an overhead view of the cell culture plate, which contains a large number of cell culture tubes arranged in an array. It is necessary to use the labelme software to label the test tube and label the cells existing in it, and the corresponding label is colony. Each image generated after annotation will generate a corresponding json file. Annotation results such as figure 2 As shown, where a is the picture before labeling; b is the picture after labeling.
[0042] (2) Establish digital image data set
[0043] Process the json file generated after labeling, divide the digital image dataset into training set, verification set and test set, and use the labelme2coco.py file to convert it into the folder structure of the coco dataset required for Mask...
PUM
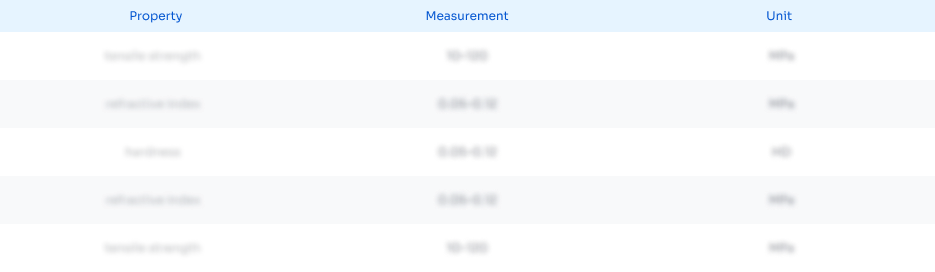
Abstract
Description
Claims
Application Information
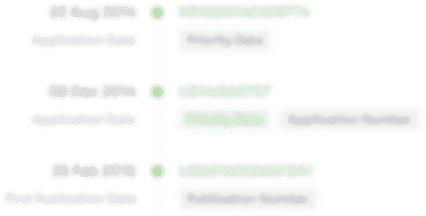
- R&D
- Intellectual Property
- Life Sciences
- Materials
- Tech Scout
- Unparalleled Data Quality
- Higher Quality Content
- 60% Fewer Hallucinations
Browse by: Latest US Patents, China's latest patents, Technical Efficacy Thesaurus, Application Domain, Technology Topic, Popular Technical Reports.
© 2025 PatSnap. All rights reserved.Legal|Privacy policy|Modern Slavery Act Transparency Statement|Sitemap|About US| Contact US: help@patsnap.com