Fine-grained Photovoltaic Load Forecasting Method Based on Temporal Convolutional Network
A convolutional network and load prediction technology, applied in the field of smart grid, can solve problems such as slow training speed, consumption of computing resources, inability to perform parallel computing, etc., to achieve the effect of flexible receptive field and small memory footprint
- Summary
- Abstract
- Description
- Claims
- Application Information
AI Technical Summary
Problems solved by technology
Method used
Image
Examples
Embodiment
[0032] Steps such as figure 1 As shown, a fine-grained photovoltaic load forecasting method based on temporal convolutional network, taking an area A such as Figure 4 The data shown from 0:00 to 23:45 on June 2, 2020 are used to predict the fine-grained photovoltaic load value at 0:00 on the 3rd. proceed as figure 2 In the steps shown, the temperature, wind force, wind speed, air pressure, and humidity are all specific values, which can be substituted into the model. The weather is cloudy to moderate rain, which is divided into two weathers, weather 1 and weather 2, and weather1 is cloudy , weather2 is moderate rain, after processing the weather data, we can get the following Figure 5 For the data shown, a total of 96 power points were collected on the 2nd, that is, N is 96. The 96 power points are spliced with the weather characteristics at 0:00 on the 3rd and used to predict the power point at 0:00 on the 3rd. The selected N can be determined according to the specifi...
PUM
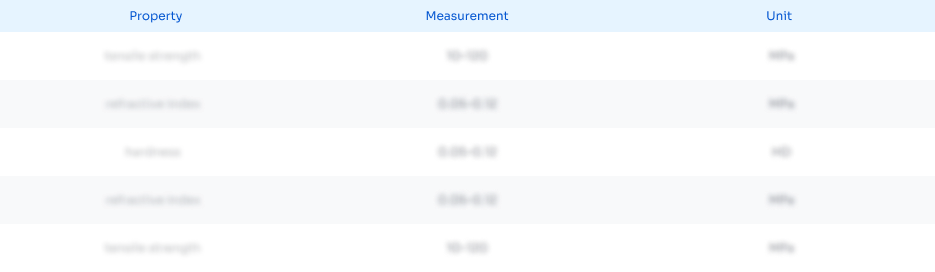
Abstract
Description
Claims
Application Information
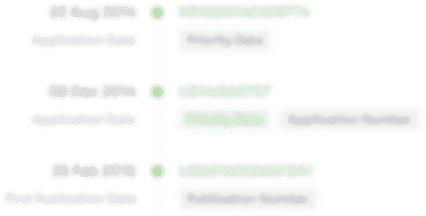
- R&D Engineer
- R&D Manager
- IP Professional
- Industry Leading Data Capabilities
- Powerful AI technology
- Patent DNA Extraction
Browse by: Latest US Patents, China's latest patents, Technical Efficacy Thesaurus, Application Domain, Technology Topic, Popular Technical Reports.
© 2024 PatSnap. All rights reserved.Legal|Privacy policy|Modern Slavery Act Transparency Statement|Sitemap|About US| Contact US: help@patsnap.com