Multi-view defense method based on noise reduction self-coding
A multi-view, self-encoding technology, applied in the computer field, achieves the effect of alleviating vulnerability and improving classification accuracy
- Summary
- Abstract
- Description
- Claims
- Application Information
AI Technical Summary
Problems solved by technology
Method used
Image
Examples
Embodiment
[0099] The following is a specific example of a multi-view adversarial autoencoder model:
[0100] Step 1: First train the multi-view deep model with the original samples, and then use the multi-view adversarial attack to generate adversarial samples to obtain a multi-view dataset containing the original samples and adversarial samples.
[0101] Step 2: Initialize the hyperparameters of the multi-view adversarial self-encoding model, input the training samples into the multi-view adversarial self-encoding model, and use the Adam optimizer to learn the model parameters.
[0102] Step 3: After training, input the test samples into the multi-view adversarial self-encoding model for pre-noise reduction processing, and the reconstructed output will be used as the input of the multi-view depth model.
[0103] The specific algorithm of model training is as follows:
[0104] 1. Construct a multi-view confrontational self-encoding model and initialize model parameters;
[0105] 2. Di...
PUM
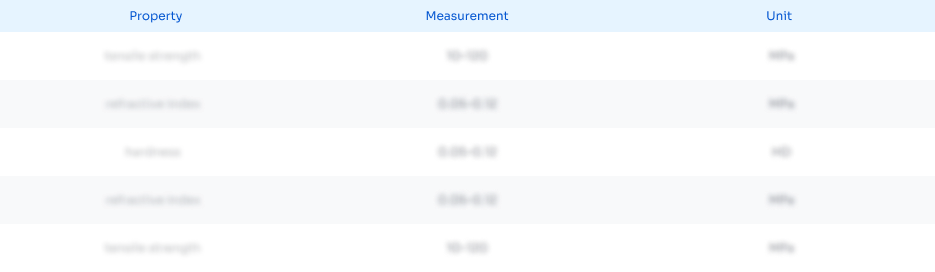
Abstract
Description
Claims
Application Information
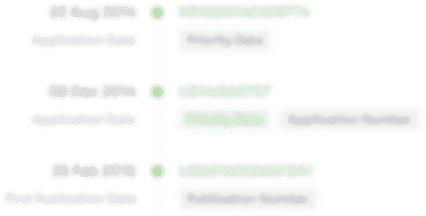
- R&D Engineer
- R&D Manager
- IP Professional
- Industry Leading Data Capabilities
- Powerful AI technology
- Patent DNA Extraction
Browse by: Latest US Patents, China's latest patents, Technical Efficacy Thesaurus, Application Domain, Technology Topic, Popular Technical Reports.
© 2024 PatSnap. All rights reserved.Legal|Privacy policy|Modern Slavery Act Transparency Statement|Sitemap|About US| Contact US: help@patsnap.com