Intelligent agent adaptive decision generation method and system based on deep reinforcement learning
A technology of reinforcement learning and generation system, which is applied in the field of agent adaptive decision generation, can solve the problems of poor stability in the training process, difficult to determine the adaptive behavior, and low robustness, so as to improve the training success rate, maintain stability, The effect of strong exploration ability and robustness
- Summary
- Abstract
- Description
- Claims
- Application Information
AI Technical Summary
Problems solved by technology
Method used
Image
Examples
Embodiment 1
[0043] This embodiment provides a method for generating an agent's adaptive decision based on deep reinforcement learning, including: obtaining historical and current environment state information, environment reward information, and decision-making action information of the agent; obtaining the environment state at the next moment information; store all the information obtained as experience in the playback buffer; train the deep reinforcement learning model of the agent; use the ordinary gradient descent optimizer to find the optimal solution of the model during the training process of the deep reinforcement learning model of the agent; The human-machine anti-interception task is used as a carrier to verify the deep reinforcement learning model of the agent.
[0044] The Soft Actor-Critic (SAC) algorithm used in this embodiment is developed based on the DDPG algorithm. It is a brand-new deep reinforcement learning algorithm that solves the high sample complexity and fragility...
Embodiment 2
[0090] This embodiment provides a method for generating an adaptive decision for an agent based on deep reinforcement learning. The difference from Embodiment 1 is that in this embodiment, the experience replay in the agent's deep reinforcement learning algorithm adopts a priority experience replay strategy.
[0091]When performing parameter updates, the Off-Policy method adopted by SAC can reuse past experience and consistently sample data from past experience, that is, experience replay (Experience Replay); the experience replay mechanism enables online reinforcement learning agents to remember and reuse Past experiences. In previous research work, experience replays were uniformly sampled from the replay buffer, however, this approach simply replays experiences with the same probability as they were originally experienced, regardless of their importance; this In the embodiment, it is considered to combine SAC with a priority experience replay (Priority Experience Replay, PER...
Embodiment 3
[0124] This embodiment provides an agent adaptive decision generation method based on deep reinforcement learning. The difference from Embodiment 1 and Embodiment 2 is that in this embodiment, the experience playback in the agent's deep reinforcement learning algorithm adopts emphasizing Recent experience playback strategy.
[0125] In Example 2, by replaying important experience more frequently, the sampling efficiency of the experience replay mechanism in the SAC algorithm is further improved, thereby speeding up the learning efficiency and convergence speed, and using the UAV anti-interception task has priority for the proposed The SAC algorithm of experience replay is tested to prove that its algorithm is effective. This embodiment adopts another method for improving the experience replay mechanism—emphasizing the recent experience replay (Emphasizing Recent Experience, ERE) strategy, and proposes the SAC+ERE algorithm.
[0126] The Emphasis on Recent Experience (ERE) repla...
PUM
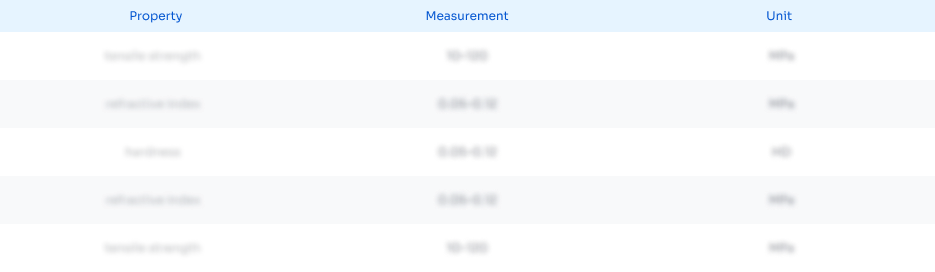
Abstract
Description
Claims
Application Information
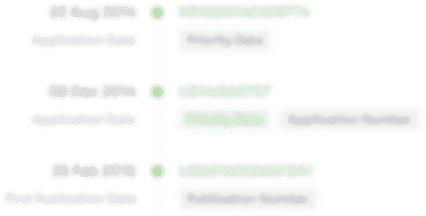
- R&D Engineer
- R&D Manager
- IP Professional
- Industry Leading Data Capabilities
- Powerful AI technology
- Patent DNA Extraction
Browse by: Latest US Patents, China's latest patents, Technical Efficacy Thesaurus, Application Domain, Technology Topic, Popular Technical Reports.
© 2024 PatSnap. All rights reserved.Legal|Privacy policy|Modern Slavery Act Transparency Statement|Sitemap|About US| Contact US: help@patsnap.com