Bridge crack detection method based on deep learning framework
A technology of deep learning and detection methods, applied in the field of image processing, can solve the problems of many noise points, high missed detection rate, complex parameter debugging, etc., to eliminate the influence of noise, alleviate the problem of missed detection, and strengthen the generalization ability.
- Summary
- Abstract
- Description
- Claims
- Application Information
AI Technical Summary
Problems solved by technology
Method used
Image
Examples
Embodiment
[0056] The program of the algorithm of this embodiment is based on the deep learning open source framework keras 2.3.1 and tensorflow-gpu2.2.0, and is developed in Python language; the hardware environment of the experiment is Intel i7 processor, NVIDIA GeForce RTX2060 graphics card.
[0057] In this embodiment, a total of 189 images with original bridge cracks were collected, and the original bridge crack images were marked with cracks to obtain a large labeled image. Synchronously cut the large label image and the original bridge crack image. After cutting, 9788 images in the training set and 6208 images in the verification set were obtained through screening, classification and amplification, both of which were 224*224 in size. Note that the image data must first be classified into the training set and the verification set before being amplified, otherwise it will cause data leakage and affect the training effect. The fracture data set is obtained after the amplification, ...
PUM
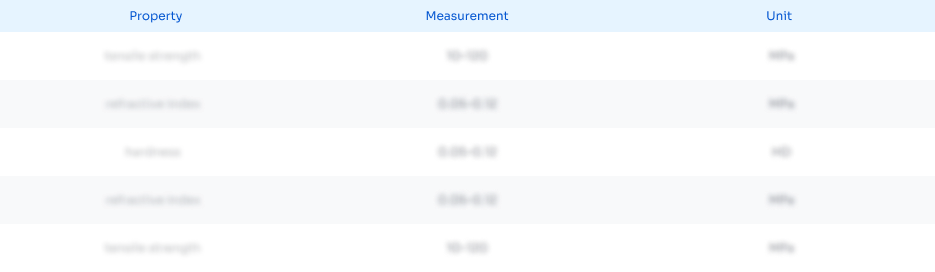
Abstract
Description
Claims
Application Information
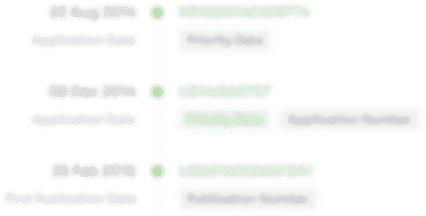
- R&D
- Intellectual Property
- Life Sciences
- Materials
- Tech Scout
- Unparalleled Data Quality
- Higher Quality Content
- 60% Fewer Hallucinations
Browse by: Latest US Patents, China's latest patents, Technical Efficacy Thesaurus, Application Domain, Technology Topic, Popular Technical Reports.
© 2025 PatSnap. All rights reserved.Legal|Privacy policy|Modern Slavery Act Transparency Statement|Sitemap|About US| Contact US: help@patsnap.com