Multi-tense remote sensing image land cover classification method based on convolutional neural network
A convolutional neural network and surface coverage technology, applied in the field of multi-temporal remote sensing image surface coverage classification, can solve the problem of low surface coverage classification accuracy, and achieve the effects of meeting the needs of automated production, improving prediction accuracy, and improving classification accuracy.
- Summary
- Abstract
- Description
- Claims
- Application Information
AI Technical Summary
Problems solved by technology
Method used
Image
Examples
Embodiment 1
[0044] Such as figure 2 The shown multitemporal remote sensing image land cover classification method based on convolutional neural network includes the following steps:
[0045] Step 1: Input the high-resolution remote sensing image data of the old and new tenses and perform corresponding preprocessing, including the production of training samples and the augmentation of training sample data; the production of the training samples includes internal refinement, field verification and There are three stages of sample cutting; the training sample data augmentation includes image data augmentation and geometric augmentation; the image data augmentation includes pixel coordinate geometric transformation data augmentation and pixel value transformation data augmentation.
[0046] Step 2: Construct a convolutional neural network, including performing feature extraction on the two temporal data input in step 1, and upsampling the high-dimensional feature map; upsampling includes dil...
PUM
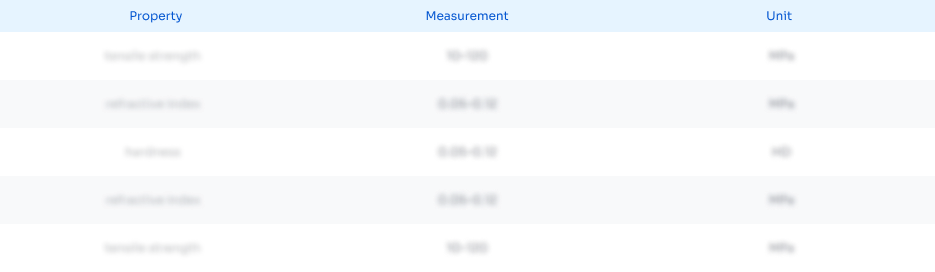
Abstract
Description
Claims
Application Information
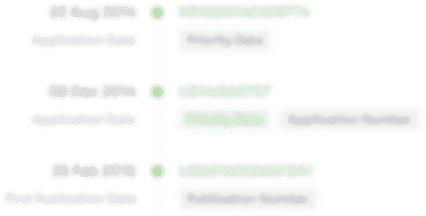
- R&D
- Intellectual Property
- Life Sciences
- Materials
- Tech Scout
- Unparalleled Data Quality
- Higher Quality Content
- 60% Fewer Hallucinations
Browse by: Latest US Patents, China's latest patents, Technical Efficacy Thesaurus, Application Domain, Technology Topic, Popular Technical Reports.
© 2025 PatSnap. All rights reserved.Legal|Privacy policy|Modern Slavery Act Transparency Statement|Sitemap|About US| Contact US: help@patsnap.com