Multi-agent cooperation model based on deep reinforcement learning
A reinforcement learning and multi-intelligence technology, applied in computing models, machine learning, computing, etc., can solve problems such as low efficiency, slow convergence, poor stability, etc., to ensure consistency, improve adaptability, and update rules.
- Summary
- Abstract
- Description
- Claims
- Application Information
AI Technical Summary
Problems solved by technology
Method used
Image
Examples
Embodiment 1
[0038] This embodiment adopts the basic structure of CCDA. The distributed Actor network is conducive to the distributed execution of agents. It interacts with the environment to generate state-action information and store it in the experience buffer. In order to combat the non-stationarity of the environment, the centralized Critic The network takes the global state-action information as input, designs the global reward R with the task of the cooperative multi-agent system as the goal, and learns a global action value Q by using TD error tot . In order to ensure the consistency between a single agent and the global optimal action, the present invention introduces the idea of value decomposition, adds the Q value decomposition network—QDN, and converts the global action value Q tot decomposes into an action value Q based on a single agent i , so that the implicit credit allocation is realized, so that the contribution of a single agent in the team can be expressed; in addit...
PUM
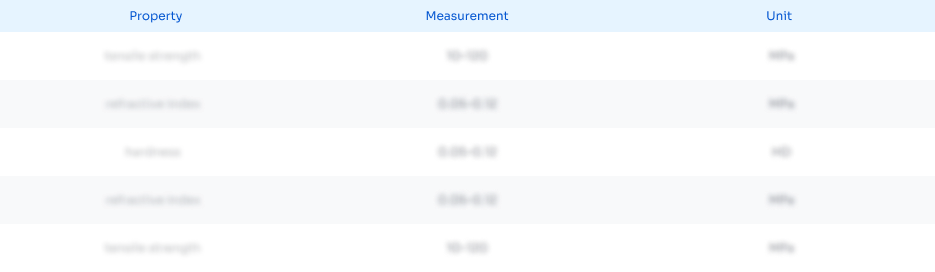
Abstract
Description
Claims
Application Information
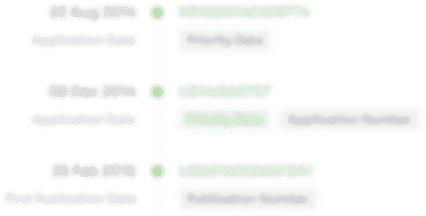
- Generate Ideas
- Intellectual Property
- Life Sciences
- Materials
- Tech Scout
- Unparalleled Data Quality
- Higher Quality Content
- 60% Fewer Hallucinations
Browse by: Latest US Patents, China's latest patents, Technical Efficacy Thesaurus, Application Domain, Technology Topic, Popular Technical Reports.
© 2025 PatSnap. All rights reserved.Legal|Privacy policy|Modern Slavery Act Transparency Statement|Sitemap|About US| Contact US: help@patsnap.com