Adversarial attack detection method
An attack detection and anti-sample technology, applied in neural learning methods, instruments, biological neural network models, etc., can solve problems such as poor performance, differences in resistance effects, and failure to take into account, so as to reduce uncertainty, stabilize results, and The effect of solving the sparsity problem
- Summary
- Abstract
- Description
- Claims
- Application Information
AI Technical Summary
Problems solved by technology
Method used
Image
Examples
Embodiment Construction
[0029] In order to enable those skilled in the art to better understand the solution of the present invention, the technical solution of the present invention will be further described below in conjunction with specific examples.
[0030] See attached figure 1 , an adversarial attack detection method, comprising the following steps:
[0031] Step S1, preprocessing the input data set of the target deep neural network to be attacked to obtain input samples.
[0032] Wherein, the preprocessing of the input data set includes the following steps:
[0033] Step S11, divide the input data set into a training set and a test set, use the training set to train the target system to predict the test set samples, remove the wrongly predicted samples, and record the rest as natural input samples.
[0034] In this embodiment, the target system to be attacked by the adversarial example is the ResNet-18 model, and ResNet-18 is composed of r 1 convolutional layers, r 2 average pooling layer...
PUM
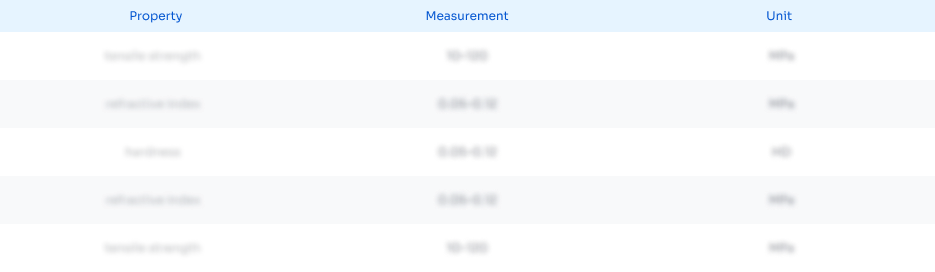
Abstract
Description
Claims
Application Information
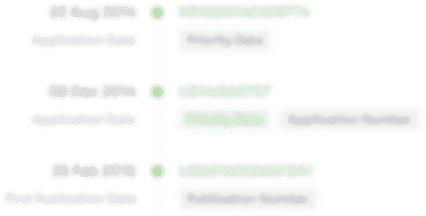
- R&D Engineer
- R&D Manager
- IP Professional
- Industry Leading Data Capabilities
- Powerful AI technology
- Patent DNA Extraction
Browse by: Latest US Patents, China's latest patents, Technical Efficacy Thesaurus, Application Domain, Technology Topic, Popular Technical Reports.
© 2024 PatSnap. All rights reserved.Legal|Privacy policy|Modern Slavery Act Transparency Statement|Sitemap|About US| Contact US: help@patsnap.com