DIDS task scheduling optimization method based on deep reinforcement learning
A task scheduling and reinforcement learning technology, applied in the field of network security, can solve the problems of DIDS load reduction, excessive state space and action space, and large memory space occupation, so as to achieve load reduction and solve the problem of excessive state space and action space Effect
- Summary
- Abstract
- Description
- Claims
- Application Information
AI Technical Summary
Problems solved by technology
Method used
Image
Examples
Embodiment Construction
[0043] The present invention will be described in detail below in combination with specific embodiments.
[0044] The DIDS task scheduling optimization method based on deep reinforcement learning of the present invention is specifically implemented according to the following steps:
[0045] Step 1. Before the work starts, evaluate the performance of each detection engine in DIDS, and collect the data volume da (unit bit) of the test traffic, detection time dt (unit ms), memory usage mu (unit Mb) and detection engine is CPU frequency Fi (unit Ghz) information, and define the calculation model of the performance index pi (performance index) of the detection engine as follows:
[0046]
[0047] After testing all the detection engines, they are divided into different grades d, d=1,...,D according to their performance. If the difference of d value is within 10%, it can be classified into the same grade;
[0048] Step 2. After starting to work, when a data packet arrives and nee...
PUM
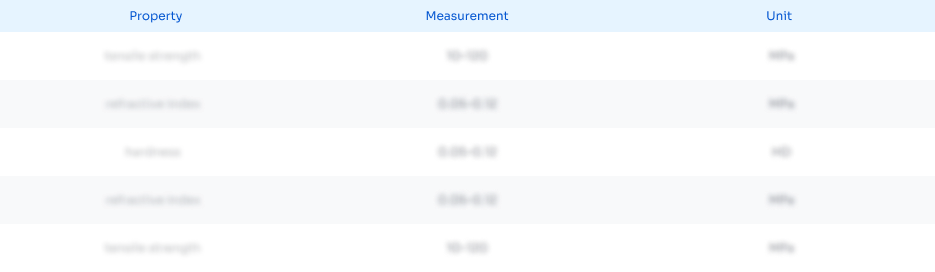
Abstract
Description
Claims
Application Information
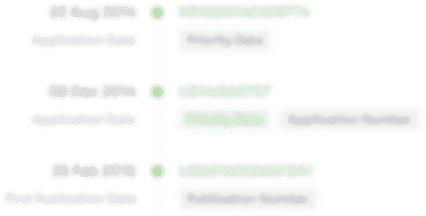
- R&D Engineer
- R&D Manager
- IP Professional
- Industry Leading Data Capabilities
- Powerful AI technology
- Patent DNA Extraction
Browse by: Latest US Patents, China's latest patents, Technical Efficacy Thesaurus, Application Domain, Technology Topic, Popular Technical Reports.
© 2024 PatSnap. All rights reserved.Legal|Privacy policy|Modern Slavery Act Transparency Statement|Sitemap|About US| Contact US: help@patsnap.com