Image super-resolution reconstruction method based on deep neural network
A deep neural network and super-resolution reconstruction technology, applied in the field of image processing, can solve the problem of losing the sensory authenticity of the image, and achieve the effect of good image authenticity, fast model convergence training speed, and improved convergence speed.
- Summary
- Abstract
- Description
- Claims
- Application Information
AI Technical Summary
Problems solved by technology
Method used
Image
Examples
Embodiment
[0050] Step 1: Dataset preparation. The most commonly used data sets in the field of image processing are: Set5, Set14, Urban100, GeneraL100, DIV2K. This training was carried out using the five datasets mentioned here. When training the General00 data set, since the GUP used at that time was only 1660S, only the size of 100×100 in the middle of the image was intercepted for reconstruction.
[0051] Step 2, for each HR image, we train LR images with amplification factors of ×2, ×3 and ×4 respectively, and the batch size is set to 64. And when training the high-power network, the output of the low-power network is used as pre-training, and the parameters of the former are used to initialize the model of the latter, which can improve efficiency and increase the quality of the results.
[0052] Step 3, using the first convolutional layer to perform feature extraction on the original input LR image. The size of each convolution kernel is 3*3, and no activation function is used t...
PUM
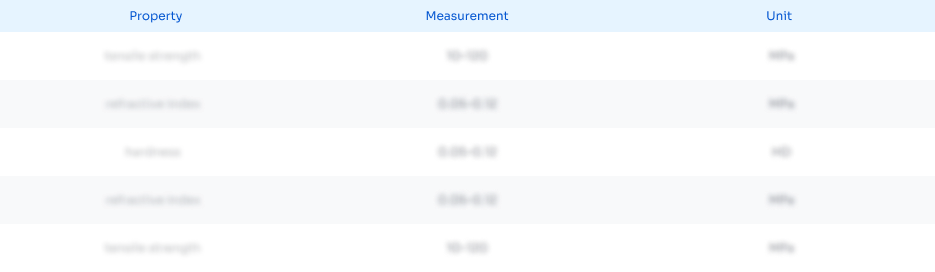
Abstract
Description
Claims
Application Information
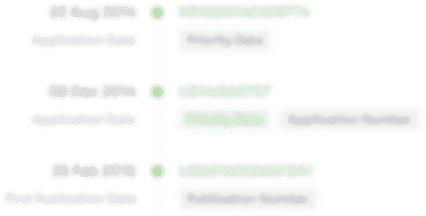
- R&D Engineer
- R&D Manager
- IP Professional
- Industry Leading Data Capabilities
- Powerful AI technology
- Patent DNA Extraction
Browse by: Latest US Patents, China's latest patents, Technical Efficacy Thesaurus, Application Domain, Technology Topic, Popular Technical Reports.
© 2024 PatSnap. All rights reserved.Legal|Privacy policy|Modern Slavery Act Transparency Statement|Sitemap|About US| Contact US: help@patsnap.com