Cross-language unsupervised classification with multi-view transfer learning
A multi-view, language technology, applied in semantic analysis, natural language translation, neural learning methods, etc., can solve problems such as harming the generalization performance of fine-tuning models and failing to capture language semantic similarity well
- Summary
- Abstract
- Description
- Claims
- Application Information
AI Technical Summary
Problems solved by technology
Method used
Image
Examples
Embodiment Construction
[0053] In the following description, for purposes of explanation, specific details are set forth in order to provide an understanding of the present disclosure. It will be apparent, however, to one skilled in the art that the present disclosure may be practiced without these details. Furthermore, those skilled in the art will appreciate that the embodiments of the present disclosure described below can be implemented in various ways, such as a process, an apparatus, a system, a device, or a method on a tangible computer readable medium.
[0054] Components or modules shown in the figures are illustrations of example embodiments of the disclosure and are intended to avoid obscuring the disclosure. It should also be understood that throughout this discussion, components may be described as separate functional units, which may include subunits, but those skilled in the art will recognize that various components, or portions thereof, may be divided into separate components or Can...
PUM
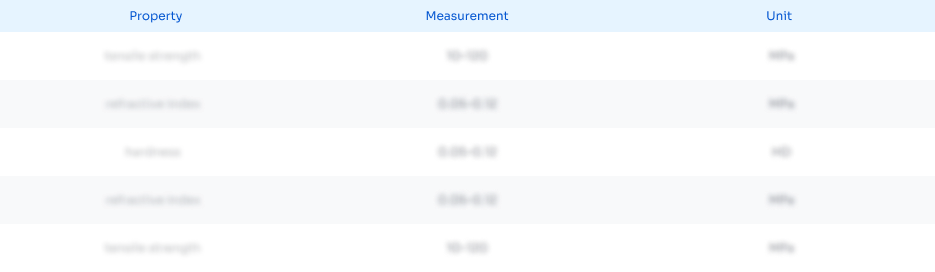
Abstract
Description
Claims
Application Information
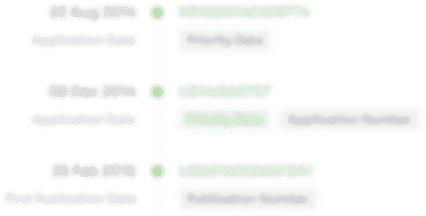
- R&D
- Intellectual Property
- Life Sciences
- Materials
- Tech Scout
- Unparalleled Data Quality
- Higher Quality Content
- 60% Fewer Hallucinations
Browse by: Latest US Patents, China's latest patents, Technical Efficacy Thesaurus, Application Domain, Technology Topic, Popular Technical Reports.
© 2025 PatSnap. All rights reserved.Legal|Privacy policy|Modern Slavery Act Transparency Statement|Sitemap|About US| Contact US: help@patsnap.com