Unsupervised machine reading understanding method based on large-scale problem self-learning
A reading comprehension and self-learning technology, applied in the field of unsupervised machine reading comprehension, can solve problems such as data difficulties and achieve the effect of improving accuracy
- Summary
- Abstract
- Description
- Claims
- Application Information
AI Technical Summary
Problems solved by technology
Method used
Image
Examples
Embodiment
[0031] Example: We use a variety of pre-trained language models (such as GPT-2 and T5) to generate a large amount of potential question and answer data from unlabeled passages of in-domain text. This method allows us to achieve cold start in a completely new domain . We then pre-train the model on these generated samples and finally fine-tune it on a specific labeled dataset.
[0032] Although a domain-specific trained model on the SQuAD1.1 training dataset achieves state-of-the-art performance (EM score of 85%) on the SQuAD1.1 Dev dataset, it is completely unable to perform the same level of inference on a completely new domain , namely NewQA (EM score of 32%). We have found that preventing overfitting on synthetic datasets is critical when pretraining models with synthetic datasets, as it often contains many noisy samples. However, these synthetic datasets are very useful when there is little or no in-domain training data in the early stage, because we can use this method ...
PUM
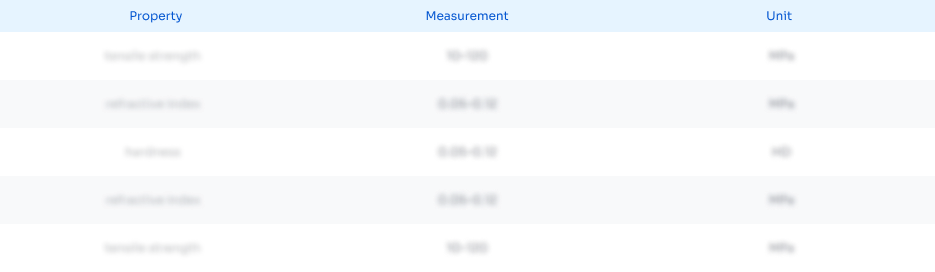
Abstract
Description
Claims
Application Information
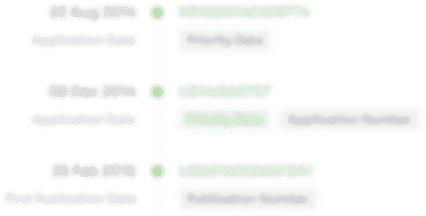
- R&D Engineer
- R&D Manager
- IP Professional
- Industry Leading Data Capabilities
- Powerful AI technology
- Patent DNA Extraction
Browse by: Latest US Patents, China's latest patents, Technical Efficacy Thesaurus, Application Domain, Technology Topic, Popular Technical Reports.
© 2024 PatSnap. All rights reserved.Legal|Privacy policy|Modern Slavery Act Transparency Statement|Sitemap|About US| Contact US: help@patsnap.com